TechnologyJuly 21, 2023
How the Industrial Edge is impacting smart manufacturing
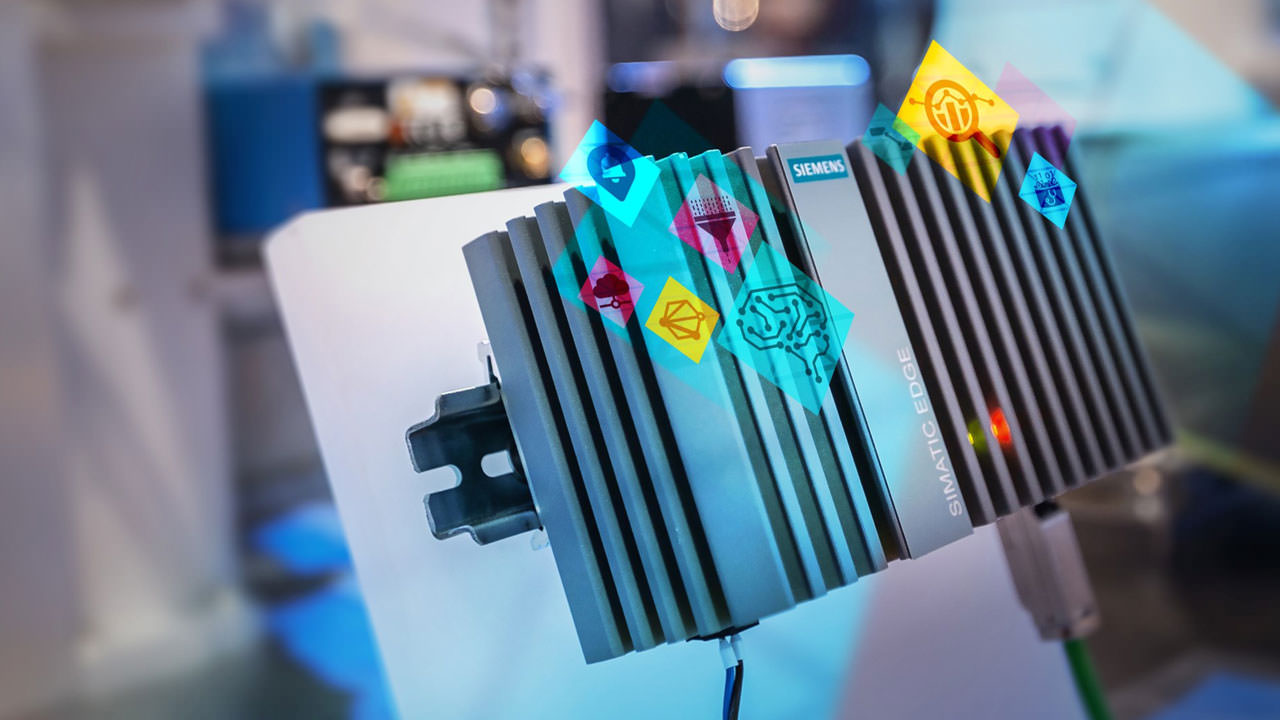
Industrial Edge and cloud computing technologies provide scalable, flexible computing resources for storing, analyzing, and visualizing the vast amounts of data industrial systems generate, and increasingly deal with cybersecurity threats. Industry experts provide their perspective on what lies ahead for industrial applications.
INDUSTRIAL EDGE AND CLOUD TECHNOLOGIES are working together to shape the future of industrial plant networks as more and more companies work to merge IT and OT operations and deal with cybersecurity threats.
In this special report, the Industrial Ethernet Book reached out to industry experts to gain their insights into the megatrends driving Industrial Edge and Cloud technology, new applications and the challenges facing automation engineers.
Edge computing with built-in cybersecurity
Leveraging MQTT, Sparkplug B to process, analyze and control data at the network’s edge.
MQTT, Sparkplug B, edge computing, and cloud applications are key technology trends for Industrial Edge and Cloud architectures, Benson Hougland, VP Product Strategy & Marketing at Opto 22 told the Industrial Ethernet Book recently.
MQTT is a messaging protocol that enables reliable real-time communication between edge devices, like sensors and actuators, and cloud-based applications. Using MQTT, these devices and applications can exchange data seamlessly.
Sparkplug B is a specification built on top of MQTT. Designed specifically for industrial automation and control systems, it provides a standardized format for publishing and subscribing to data. This format ensures interoperability and easy integration between devices and applications.
“Industrial Edge architectures use edge computing devices with built-in cybersecurity features to process, analyze, and control data where it’s produced, at the network’s edge. Local processing improves data quality and reduces the amount of data sent to the cloud, for faster response times and improved connectivity,” Hougland said.
“Cloud applications provide scalable, flexible computing resources for storing, analyzing, and visualizing the vast amounts of data industrial systems generate. Cloud applications can offer advanced analytics, machine learning, predictive maintenance, HMIs for remote monitoring and supervisory control (SCADA), and much more,” he added.
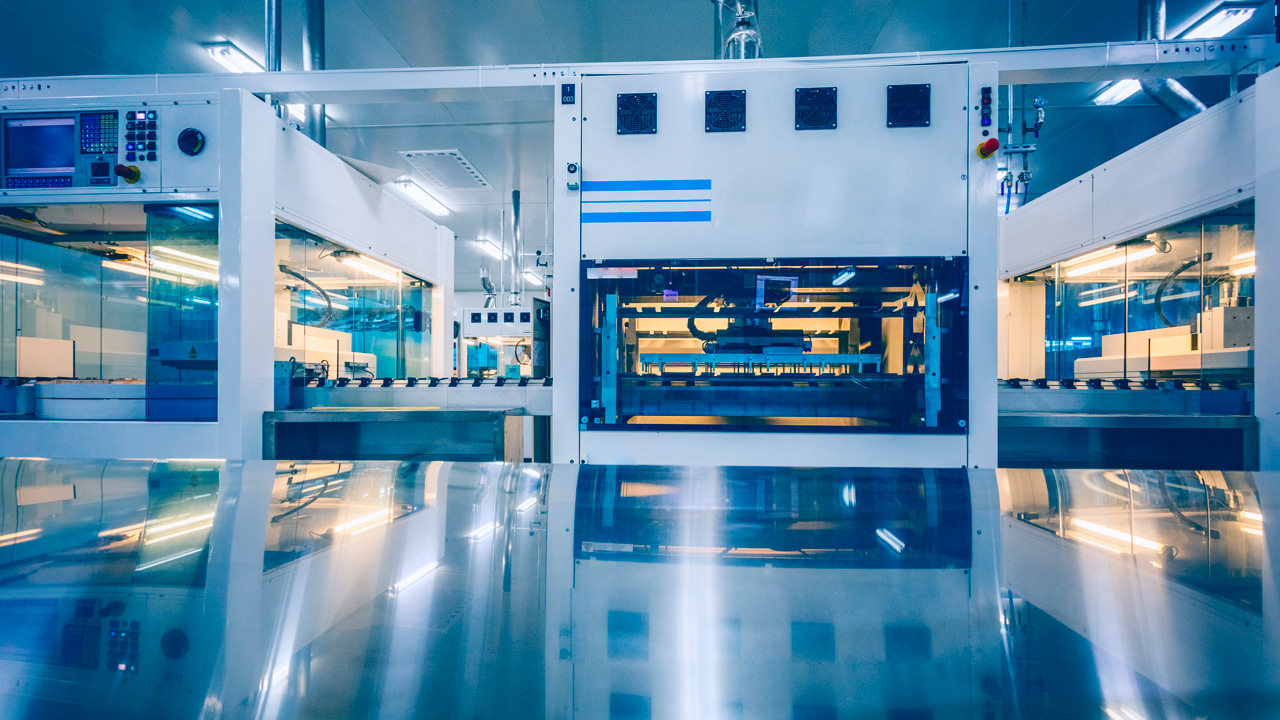
“Industrial Edge architectures use edge computing devices with built-in cybersecurity features to process, analyze, and control data where it’s produced, at the network’s edge. Local processing improves data quality and reduces the amount of data sent to the cloud, for faster response times and improved connectivity,” — Benson Hougland, VP Product Strategy & Marketing, Opto 22.
Looking at the benefits
For manufacturers, industrial edge and cloud computing offer cybersecurity, data democratization, scalability, and easier ways to manage industrial systems.
Industrial systems are often vulnerable to cyber threats, making robust cybersecurity measures critical. Edge and cloud architectures employ secure communication protocols, data encryption, access control, and authentication mechanisms that help protect industrial infrastructure, data, and operations from unauthorized access and data breaches. It is critical that these cybersecurity measures are employed at both the edge and at the cloud layers.
“Edge and cloud architectures democratize OT data, making it accessible to stakeholders across the organization,” Hougland said. “Centralizing data storage and analysis in the cloud means that authorized personnel can access real-time and historical data from anywhere, improving decision-making, optimizing performance, and making operations more efficient.”
Data volume constantly grows, and operational requirements expand, but industrial edge and cloud architectures can easily scale to accommodate them. Cloud computing provides virtually unlimited storage and computing resources. Edge computing distributes data processing to handle increasing workloads.
Hougland added that industrial edge and cloud architectures also make it easier to manage industrial systems. Edge computing offers local management and control for a granular level of oversight and autonomy in industrial environments. Meanwhile, cloud applications centralize management and monitoring, so administrators can oversee multiple edge devices, applications, and data streams from a single interface. This centralized management simplifies system configuration, updates, and maintenance, reducing operational overhead and improving overall system reliability.
Importance of cybersecurity
In industrial environments, cybersecurity is critical. Communication technologies like MQTT and TLS (transport layer security) address cybersecurity concerns in industrial edge-to-cloud architectures because they are edge-originated. Secure communications originate in the edge device, with no open inbound firewall ports needed to transport data. Edge devices publish their data outbound only, and software applications subscribe to this data using the same secure methods.
Hougland said that the key to democratizing operational data is reducing the tight coupling between data-generating devices and the software applications that need the data. MQTT provides this decoupled architecture: all data messages are brokered through a server. Many publishers can send their data to the broker, many subscribers can choose the data they need from what is published, and all can work with the data simultaneously.
In addition, this broker-based architecture provides a single point to manage all data participants and access to the data, from both access control and communication channel standpoints.
Applications focus
Hougland said that edge-to-cloud architectures are obviously well suited for industrial applications that are geographically dispersed, such as Oil & Gas, Water/Wastewater, and other remote monitoring applications.
But manufacturers are widely implementing these architectures as well. In particular, manufacturers find that Operational Equipment Effectiveness (OEE) can benefit from industrial edge and cloud solutions, where accessibility to machine status and production counts can help drive digital transformation initiatives.
OEE data can benefit plant-floor personnel who operate manufacturing equipment locally at the edge. It can also provide valuable company-wide information to stakeholders such as finance and sales operations, whether on-premises or in the cloud. This information can drive efficiencies and aid in planning across the entire organization.
“Increasingly, automation engineers are faced with the expectation that operational data should be available for immediate use in corporate software applications,” Hougland said. “Yet these same engineers must keep all operations running safely and securely without interruption. And they often have proprietary or legacy systems whose data is locked inside, making data access difficult and costly.”
“Edge computing and cloud computing, however, give automation engineers powerful solutions to address these security, scalability, and OT data accessibility challenges,” he added.
Edge computing enhances security by processing data locally, minimizing exposure to cyber threats. It also enables local decision-making and control, improving scalability and responsiveness. Cloud computing complements edge security measures with robust centralized security protocols.
It offers virtually unlimited storage and computing resources, allowing engineers to scale applications and data storage as needed.
Edge computing also provides local accessibility to real-time data, so engineers and operators can make faster decisions. Cloud computing, on the other hand, offers centralized access to OT data from anywhere for comprehensive analysis and informed decision-making. With advanced analytics and visualization tools in the cloud, engineers gain valuable insights for process optimization.
“This combination of edge and cloud computing lets automation engineers adopt a multi-layered security approach, achieve scalability for increasing workloads, and enhance accessibility to OT data. By leveraging these advancements, engineers can design resilient architectures that address key challenges and unlock the full potential of industrial automation data,” Hougland said.
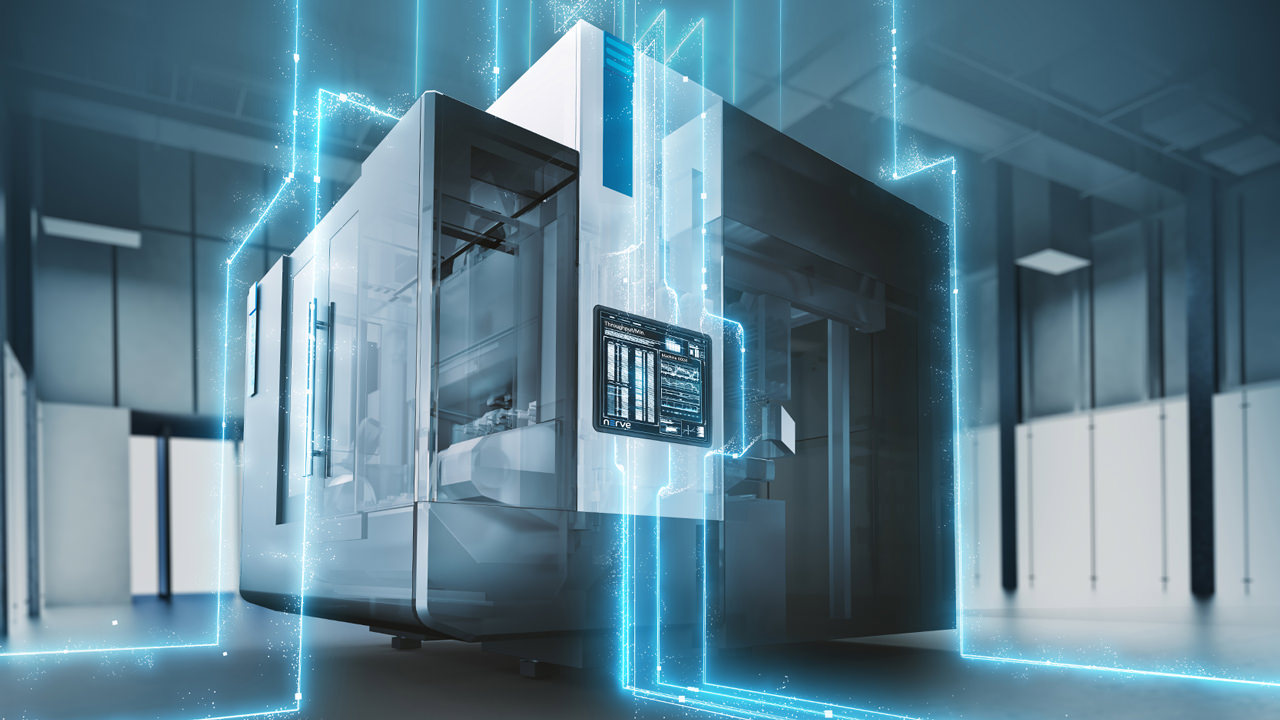
“The integration of artificial intelligence (AI) and machine learning (ML) algorithms is a key trend enabling the emergence of industrial edge and cloud technologies. AI and ML empower industrial systems to learn from data, derive actionable insights, and make intelligent decisions.” — Georg Stöger, Director Training & Consulting, TTTech Industrial.
Impact of IoT real-time data collection
Shift of data processing requirements from the cloud to the industrial edge.
According to Georg Stöger, Director Training & Consulting at TTTech Industrial, the emergence of industrial edge and cloud technologies is fueled by several key technology trends that are revolutionizing the industrial landscape. One trend is the widespread adoption of Internet of Things (IoT) devices in industrial settings and the shift of data processing requirements from the cloud to the industrial edge.
“IoT devices provide real-time data collection, enabling enhanced visibility and control over industrial processes. These devices, such as sensors and actuators, connect the physical and digital worlds, facilitating data-driven decision-making,” Stöger said. “Accordingly, we observe advancements in edge computing capabilities. Edge computing brings data processing and analysis closer to the source, reducing latency and enabling faster response times. This trend is particularly crucial in industrial settings where real-time decision-making is critical.”
“Nevertheless, cloud technologies continue to play a vital role in the industrial edge ecosystem. Cloud platforms provide scalable and flexible computing resources, centralized data storage, and advanced analytics capabilities. They enable seamless integration, data sharing, and remote management of industrial systems, Stöger added.
AI and ML algorithms
Stöger said that the integration of artificial intelligence (AI) and machine learning (ML) algorithms is a key trend enabling the emergence of industrial edge and cloud technologies. AI and ML empower industrial systems to learn from data, derive actionable insights, and make intelligent decisions.
In summary, the key technology trends enabling the emergence of industrial edge and cloud technologies include IoT adoption, advancements in edge computing, the utilization of cloud platforms, and the integration of AI/ML. TTTech Industrial capitalizes on these trends to deliver cutting-edge industrial solutions that drive efficiency, productivity, and innovation.
The combination of industrial edge and cloud computing also offers several very relevant benefits in manufacturing from both a technical and a commercial viewpoint.
“Industrial edge computing brings data processing and analysis closer to the source, enabling real-time decision-making and reducing latency. This allows for faster response times, improved operational efficiency, and enhanced productivity. Edge computing also facilitates local data storage and processing, ensuring data security and privacy, for example in situations where some edge-local data should not be accessible from the cloud, or needs to be pseudonymized before being uploaded to the cloud,” Stöger said.
“Cloud computing, on the other hand, provides scalable and flexible computing resources. It enables centralized data storage, advanced analytics, and remote management of industrial systems. Cloud platforms offer the ability to handle large volumes of data, perform complex computations, and provide real-time insights. They also enable seamless collaboration and data sharing across different locations,” he added.
In combination, industrial edge and cloud technologies provide manufacturers with real-time visibility into production processes, enabling proactive maintenance, quality optimization, and resource allocation. They facilitate predictive analytics, enable predictive maintenance, and help to reduce downtime. Manufacturers can also leverage the cloud for remote monitoring and control, facilitating more agile and flexible production processes.
To summarize, the combination of industrial edge and cloud computing provides technical benefits such as real-time decision-making, scalability, data security, and advanced analytics. Its potential impact on manufacturing includes improved efficiency, productivity, quality, and flexibility, ultimately driving digital transformation in the industry.
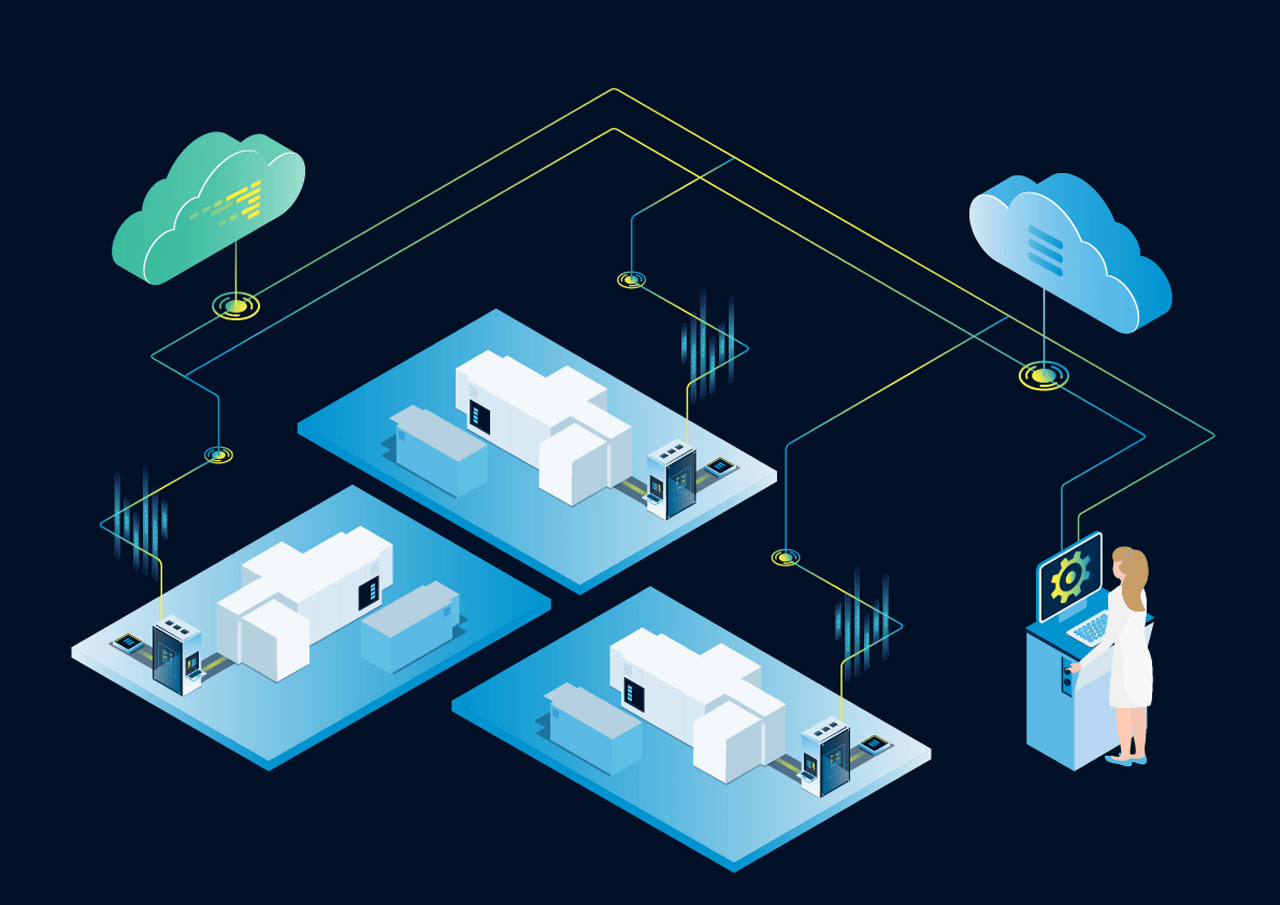
An edge computing platform like TTTech Industrial’s Nerve enables secure connectivity between machines and production locations, as well as remote access and remote management of data, applications, and software.
Cloud-based Nerve platform
TTTech Industrial’s cloud-based edge computing platform, Nerve, offers unique technical capabilities that set it apart in the industrial landscape. Nerve combines the power of edge computing and cloud technologies with open interfaces to access and process data in the OT domains, host all kinds of user-specific applications at the edge, and provide seamless connectivity, real-time analytics, and remote management for industrial applications.
What makes Nerve unique is its ability to ingest, process, and analyze OT data including real-time fieldbus data robustly and securely directly at the edge, close to the source, while also leveraging the scalability and flexibility of the cloud. It provides a distributed architecture that enables field devices, such as sensors and machines, to collect and process data locally, reducing latency and enabling faster response times. Nerve then seamlessly integrates with the cloud, allowing for centralized data storage, advanced analytics, and remote monitoring and control.
This technology finds application in various industrial scenarios. For example, in manufacturing, Nerve enables real-time monitoring of production lines, predictive maintenance, and quality optimization. It facilitates the collection and analysis of data from diverse industrial assets, enabling actionable insights for operational improvements. Nerve’s edge computing capabilities enable local decision-making, reducing dependence on cloud connectivity and ensuring continuity in critical operations.
Furthermore, Nerve’s cloud-based management system allows for secure data storage, efficient collaboration, and scalability. It provides a centralized platform for remote management, enabling organizations to monitor and control industrial processes from anywhere. Nerve easily connects to advanced analytics capabilities provided by various hyperscaler cloud offerings, supporting the development and deployment of intelligent applications e.g., for anomaly detection and predictive analytics.
TTTech Industrial’s Nerve platform stands out by combining edge computing and cloud technologies, offering secure connectivity, real-time analytics, and remote management for industrial applications. Its unique features enhance operational efficiency, enable predictive maintenance, and facilitate the development of intelligent industrial solutions.
Industrial edge and cloud solutions
“The newest industrial edge and cloud solutions are targeting specific application areas across various industries. One prominent area is manufacturing, where these solutions enable real-time monitoring of production lines, predictive maintenance, quality optimization, and resource allocation. They empower manufacturers with data-driven insights, improving efficiency and productivity,” Stöger said.
He added that another key application area is energy and utilities, where industrial edge and cloud solutions support smart grid management, energy optimization, and asset monitoring. These solutions enable real-time analysis of energy consumption patterns, predictive maintenance of infrastructure, and optimization of energy distribution.
In transportation, industrial edge and cloud solutions facilitate fleet management, route optimization, and vehicle health monitoring. They enable real-time tracking and analysis of data from vehicles, contributing to operational efficiency and enhancing safety.
These solutions play a significant role in the broader Internet of Things (IoT) and enterprise connectivity landscape. Leveraging industrial edge computing enables data processing and analytics to occur closer to the data source, reducing latency and enabling real-time insights. The cloud aspect provides scalable and flexible computing resources, centralized data storage, and advanced analytics capabilities. This integration of edge and cloud technologies ensures seamless connectivity, enabling IoT devices and enterprise systems to communicate and share data efficiently.
Overall, the newest industrial edge and cloud solutions are targeting application areas such as manufacturing, energy, and transportation. They contribute to IoT and enterprise connectivity by enabling real-time insights, optimizing operations, and facilitating seamless data exchange between devices and enterprise systems.
“Advances in edge computing and cloud computing address several challenges that automation engineers face in their work. One key challenge is the increasing complexity and scale of industrial automation systems. With the integration of edge computing, automation engineers can distribute computing resources and processing capabilities closer to the edge devices, reducing the burden on central control systems and improving system performance and responsiveness,” Stöger said.
Another challenge is the need for real-time decision-making and data analysis. Edge computing enables automation engineers to process and analyze data locally at the edge devices, enabling faster response times and real-time insights. This is particularly crucial in time-sensitive industrial processes.
Cloud computing addresses challenges related to data storage, scalability, and collaboration. Automation engineers can leverage cloud platforms to securely store and manage vast amounts of industrial data, eliminating the need for on-premises infrastructure. Cloud-based solutions also provide scalability, allowing for the expansion of computing resources based on demand.
Furthermore, cloud platforms facilitate seamless collaboration and data sharing, enabling automation engineers to work together efficiently and access real-time insights from anywhere.
“Overall, the advances in edge computing and cloud computing help automation engineers overcome challenges related to system complexity, real-time decision-making, data storage, scalability, and collaboration, enabling them to design, deploy, and manage automation systems more effectively,” Stöger said.
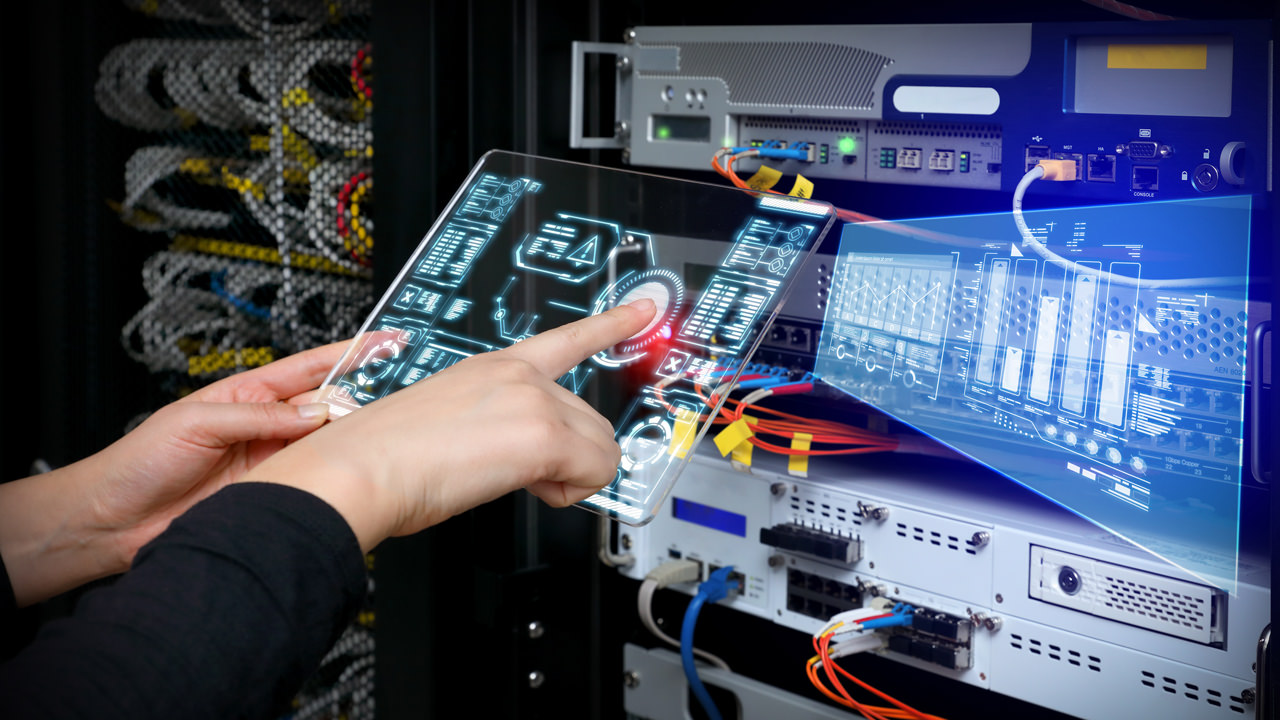
“By combining industrial edge technology with cloud infrastructure, an organization can access real-time, actionable data. This is achieved through bidirectional communication with the industrial application, executed securely, swiftly, and easily. ” — Barry Turner, Technical Business Development, Red Lion.
Real-time, actionable data
Local edge computing and cloud transfers for long-term storage, analytics, AI, machine learning, and visualization.
According to Barry Turner, Technical Business Development at Red Lion, “Industrial edge IoT devices capable of capturing data directly from virtually any element within the plant floor, locally processing it, and securely transmitting the output to a cloud provider via MQTT, has dramatically transformed the industrial sector. Now, organizations have the capability to access superior real-time data through the combination of local edge computing and cloud transfers for long-term storage, analytics, AI, machine learning, and visualization.”
“Moreover, there is a growing trend among industrial users towards harmonizing their security policies with those established by the IT department. As the connectivity of industrial devices to the internet expands, the necessity of applying robust security measures to safeguard these critical assets becomes paramount. The implementation of cybersecurity policies that are in sync with those of the IT department represents the gold standard approach in securing industrial applications,” Turner added.
By combining industrial edge technology with cloud infrastructure, an organization can access real-time, actionable data. This is achieved through bidirectional communication with the industrial application, executed securely, swiftly, and easily. One significant challenge within the realm of IIoT application is the so-called ‘first mile’ – capturing data directly from the industrial application’s components.
“Red Lion simplifies this crucial step by offering a solution capable of directly interfacing with almost any component within the plant floor or other industrial applications. Additionally, local computation becomes a necessity when the data is originating from multiple diverse sources. Red Lion’s Crimson software and industrial hardware platforms, like FlexEdge or Graphite, function as excellent edge devices. They’re able to access diverse data device types, perform edge computation, and then securely transmit the data to its intended destination. Often, this implies sending data to the cloud for storage, analysis, visualization, and AI application,” Turner said.
OT devices at core of innovation
Turner said that connecting the output of OT devices directly to an organization’s cloud solution is the core of innovation. It’s through this direct link that we glean insights on how to enhance processes, increase efficiency, and maximize productivity from our industrial machinery.
“Various protocols can be employed to link applications to the internet or the cloud. However, MQTT has emerged as the protocol that meets the need of the majority of industrial application demands when transmitting data via an internet connection. Its efficiency, security, and user-friendly nature make it an ideal choice. Unlike the traditional poll-response method, which continuously checks devices for data changes, MQTT allows applications to ‘report by exception,’ which means data is written only when changes occur,” he said.
He added that the efficiency of MQTT is not confined to this feature. It also enables devices to subscribe and publish data to specific devices, allowing for tailored data interaction. A device can be configured to subscribe only to the data that is crucial for it, avoiding unnecessary information overload. Security, a vital aspect of any industrial application, is another area where MQTT excels. It facilitates encrypted, authenticated access to your data, making it an ideal fit for IIoT applications.
“As the connectivity of industrial devices expands, adhering to reliable protocols like MQTT is becoming increasingly vital. Its seamless integration with established IT security measures makes it a gold standard in securing industrial applications,” Turner said.
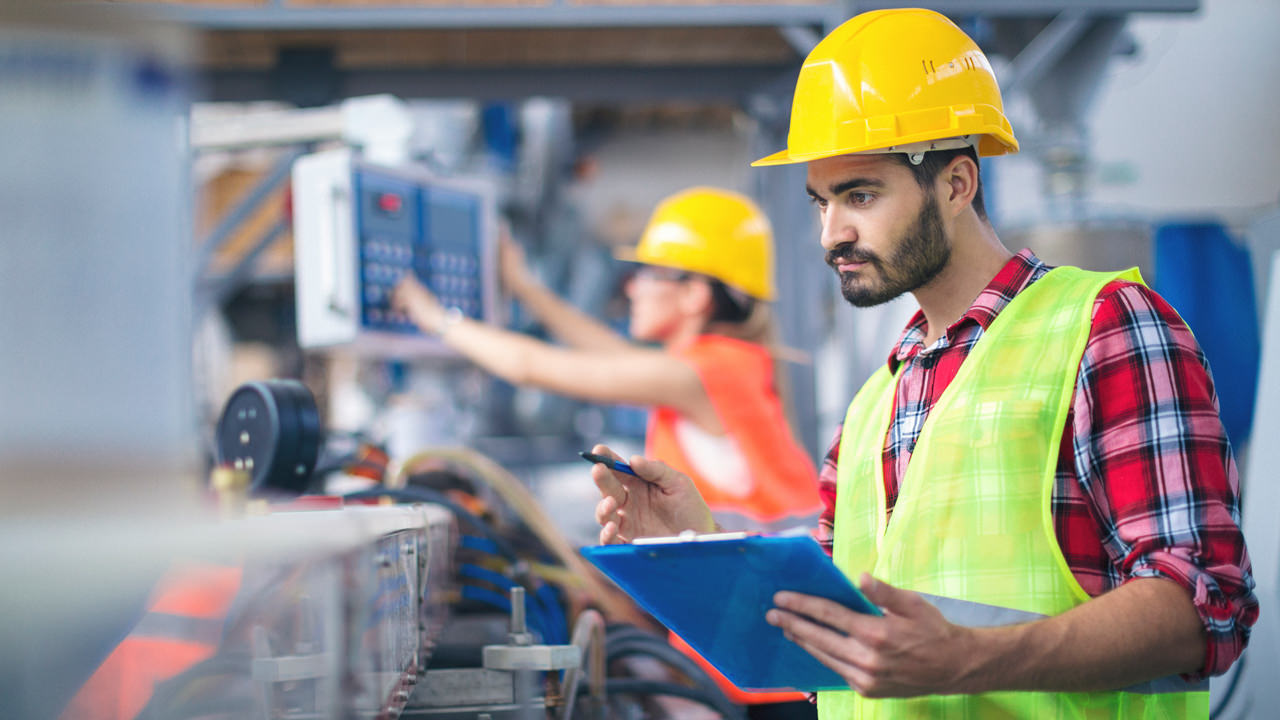
“Once data has reached the edge or the cloud, AI related technologies such as machine learning can increase the effectiveness of traditional approaches to quality such as machine vision. Machine learning can allow an end user to create a defect identification algorithm with boundary parameters and example images to identify defects,” Dr. Al Beydoun, President and Executive Director, ODVA.
Leveraging fast, flexible Ethernet communications
Focus on quality and AI-related technologies such as machine learning.
According to Dr. Al Beydoun, ODVA President and Executive Director, key technology trends are enabling the emergence of Industrial Edge and Cloud technologies.
“Increased Ethernet bandwidth and flexible wireless communication such as Wi-Fi or 5G along with multilayer security, labelled data, and AI related technologies including machine learning are key contributors to the adoption of edge and cloud computing in industrial automation, Beydoun told the Industrial Ethernet Book recently. “Fast and flexible Ethernet is required to transport data from the plant floor to the edge and/or cloud for analysis and then back to optimize a process without causing any disruptions.”
“Security is also another critical factor in allowing key factory information to be transported beyond the PLC to either an edge appliance or outside the plant gate to the cloud,” he added. “Without the ability to authenticate, encrypt, and prevent tampering of data it would be far too risky to rely on any type of computing that is external to the core automation system. Additionally, it’s critical that the data includes proper context that a data model can provide to ensure that the information can be best taken advantage of to improve operations.”
Beydoun said that, once data has reached the edge or the cloud, AI related technologies such as machine learning can increase the effectiveness of traditional approaches to quality such as machine vision. Machine learning can allow an end user to create a defect identification algorithm with boundary parameters and example images to identify defects. An iterative process then takes place with an operator reviewing the accuracy of the training model based on the initial guidelines and base training set images to identify opportunities for improvement. From there, the machine learning vendor can help tweak the algorithm and parameters to help identify hard to identify errors. After that, the machine learning model will improve as additional data is ingested through the operation of the process.
EtherNet/IP and the cloud
EtherNet/IP is an industrial network that can enable the usage of edge and cloud applications to improve operations. It does this through its physical layer independence, allowing for the use of fast Ethernet, Wi-Fi, and 5G, as well as support for a range of properly labeled data, including the ability to use process device profiles, the Process Automation Device Information Model (PA-DIM), and the OPC UA companion specification. Additionally, EtherNet/IP is based on standard TCP/IP Ethernet making the data easily routable to where it needs to be, and EtherNet/IP is lightweight and fast enough to ensure the data arrives when it needs to get there. EtherNet/IP also supports device level security as a part of a defense in depth approach with CIP Security.
Industrial edge computing
Beydoun said that industrial edge computing enables an on-premise solution that can help to lessen security risks by reducing physical access as well as potential external network access. Additionally, edge computing is a fixed capital expenditure that isn’t at risk of increased fees over time. Edge appliances can also offer increased computing speeds due to physical proximity for highly critical processes.
Cloud computing, on the other hand, is an ongoing subscription cost, versus a capital investment, that is dependent on the amount of data stored and services utilized. However, the cloud does offer the benefit of data being stored off site to reduce the risk of loss through fire or other damage to the physical hardware. Additionally, if the data includes the proper contextual and description information then it can be easily manipulated in the cloud by the latest algorithms and business analysis tools. This advantage can increase over time as new analytic technologies such as those powered by AI become available.
“The differences in the costs and benefits of the technologies suggest different approaches depending on applications, market segments, cost structures, and market conditions,” Beydoun said.
“However, what is clear is that a hierarchical model can be created with the most critical operations being confined to a robust PLC to ensure that if all else fails that the process will keep running and that security, safety, and sustainability risks will be minimized. From there, the amount and types of data that will be transported to either an edge appliance or to the cloud is dependent on how quickly and reliably the data needs to be transferred, what security concerns there are, and how large of a business benefit there is to being able to take advantage of the newest cloud analysis software for process optimization.”
Edge and cloud processing
Edge and cloud processing enables applications that weren’t possible before such as chemical reactions that require constant intervention to flow of various base inputs to keep a process under control. This is particularly valuable for applications that are very sensitive to ambient temperature changes and feedstock quality disparities. This opens the door to operations that recycle previously used materials or byproducts from other processes that have large variations from batch to batch.
Ultimately, the idea of AI and machine learning models is to take the advantage that humans have of being able to accurately identify common issues along with the ability to deal with new and unique problems and to build that capability into a software tool. Machine learning can be relatively simple with identifying parameters that identify an object or a deviation from the norm that are built into a model that can then improve over time with additional data just as a human would. This is a more rigid approach with less opportunities to learn, but with a tighter adherence to the prescriptive boundaries applied.
Deep learning, a subset of machine learning, on the other hand relies on complex patterns in the image versus set parameters. Deep learning matches the image to the outcome and uses large amounts of data to get more accurate. The advantage to deep learning is that it can be better at identifying variations of an issue that don’t conform to the typically identified parameters, which can contribute to greater accuracy and less manual work of identifying parameters. However, deep learning requires fast processing capabilities and a very large data set to be effective. Additionally, deep learning model problems are hard to troubleshoot since parameters aren’t manually controlled.
Focus on machine learning
Beydoun said that quality presents a significant opportunity for machine learning to be applied to existing vision systems. Machine learning for quality applications is particularly valuable when looking at hard use cases such as label printing errors and foreign material contamination in consumer-packaged goods or weld spatter than could indicate a durability concern on an automotive line. Additionally, for a chemical plant, algorithms can be implemented to optimize closed loops that ensure close adherence to set points regardless of external conditions.
Regardless of the application or industry, edge and cloud solutions share the same advanced, automated data processing that can help to improve operations. The value that edge and cloud analytics can provide to industrial automation offers a significant incentive to build out the proper automation infrastructure including properly designed network zones and conduits that separate out different automation areas with advanced switches to improve both network performance and security, data that is contextualized and ready for transport, and security to ensure that two way data transfer doesn’t allow a bad actor an easy opportunity to disrupt operations.
Challenges for automation engineers
Beydoun said that “unfortunately controls engineers don’t have the time to continuously revisit already implemented solutions. Additionally, operational excellence workers are already inundated with more projects than they can handle. Setting up processes to optimize themselves and to be able to handle new scenarios is a great advantage to lean organizations.”
Edge and cloud computing that can help identify quality issues are especially important as raw material inputs and feedstocks can vary and change slightly over time. A system that can adjust automatically to new information, even if the algorithm just identifies new anomalies that a worker can then manually check to confirm whether there is an issue or not, can be the difference between a widespread recall or a few hours of downtime adjusting to a previously unknown or unidentified concern.
Data tracking and optimization through the edge and cloud can also be applied to maintenance concerns. Tracking the time to return of a pneumatic cylinder or the accuracy of a weld over time can help to identify when an automation device needs to be replaced on a more accurate schedule to reduce waste and downtime as well as to provide ample warning time to prepare for the changeover.
It’s important to start by making a plan for what optimization loops make sense to be created to solve existing business problems. This will help to identify the business returns that will help justify the investment required to prepare for the future. Ultimately, setting up a tiered PLC, edge, and cloud solution with multilayered security with ordered and identified data will make it possible for extremely fast processing at the edge and highly flexible analysis in the cloud to optimize production across a wide variety of parameters. Lightweight proven control networks such as EtherNet/IP that are working to provide contextualized data through data models like OPC UA and PA-DIM combined with defense in depth security solutions such as CIP Security device level security will help to enable this advanced architecture for the future.

Functionalities of the Edge PCs from Phoenix Contact.
System interoperability
Importance of openness and no proprietary technologies, interfaces or protocols.
“The essential key component to be able to successfully use edge and cloud computing in production in the manufacturing industry is interoperability between the systems and the possibility to use desired applications on different targets,” said Daniel Korte, Technology Manager PLCnext Technology for Phoenix Contact Electronics GmbH in Bad Pyrmont, Germany.
“For this purpose, the systems used should use openness as the leading variable and no proprietary technologies, interfaces or protocols should be used. OCI containers and virtualization in general can be named as key technologies here as a basis for the use of applications that is as hardware-independent as possible. In addition, the deployment of these applications must function via standard mechanisms. Other key technologies and topics are security and real-time (cloud) communication through the use of TSN, e.g. Profinet via TSN,” Korte said.
Edge and cloud computing
Korte said that the major goal to be achieved by the joint use of edge and cloud computing is to obtain added value from data. This can be cost reductions as well as productivity increases or improved quality. Ultimately, there must be an economic benefit for the user.
Specifically, the use of edge computing is close to the data source, which leads to lower latencies and, in part, to better data protection, since the data is here in the local network. The user also has the issue of security directly in hand here. The edge devices are suitable for data (pre)processing, data buffers, data collection and the use of AI is also conceivable.
The cloud is, of course, ideal for storing and processing large amounts of data and for learning AI models. The cloud resources are scalable and available worldwide. The topic of security is also very important here but is usually solved directly by the cloud operator.
Applications focus
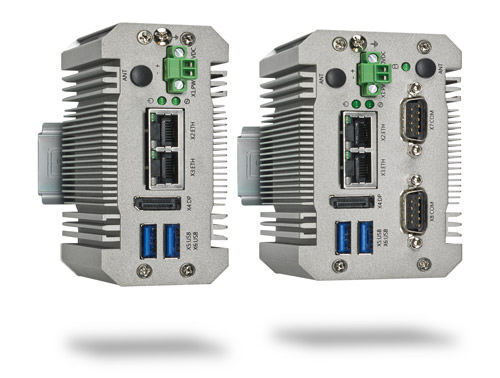
With preinstalled software packages as well as universal graphical user interfaces and programming environments, the powerful hardware of the Edge PCs from Phoenix Contact enables simple development and implementation of edge applications.
“The specific applications for edge and cloud consulting in the industrial context are classic connectivity and data infrastructure applications. In edge computing, these include above all the brownfield connection of machines in order to leverage the added value of the data without exchanging the PLC or accessing the logic of the machine,” Korte added. “This is mainly about connectivity with data buffers and data pre-processing. Of course, new machines are also equipped with the possibilities of the Edge, here the applications can be executed both on a separate Edge Device and directly on the PLC, as in our case with PLCnext Technology.”
He said that the typical use cases of the cloud here are data visualization using dashboards and optimization of the machine. For optimization, both statistical methods and artificial intelligence can be used, e.g. for predictive maintenance or, as with Proficloud.io, predictive energy. In general, smart services play a major role here.
One of the greatest challenges for automation engineers is the convergence of information technology and operation technology. Here, the networks are growing together and it should be possible to access and, if necessary, even influence the machine data by means of IT-typical standards. This poses a great challenge to classic automation technology, since a simple connection to IT systems is not readily possible here. However, this is crucial because the availability of data as well as cybersecurity are a basic requirement for the successful use of edge and cloud computing.
“The open architecture of PLCnext Technology makes it possible for automation engineers to either install edge applications on the Linux system or as OCI containers on their own, or simply use existing apps by leveraging the PLCnext Store. This is crucial as not everyone has in-depth Linux know-how. Thus, data availability is no longer a major challenge here,” Korte added.
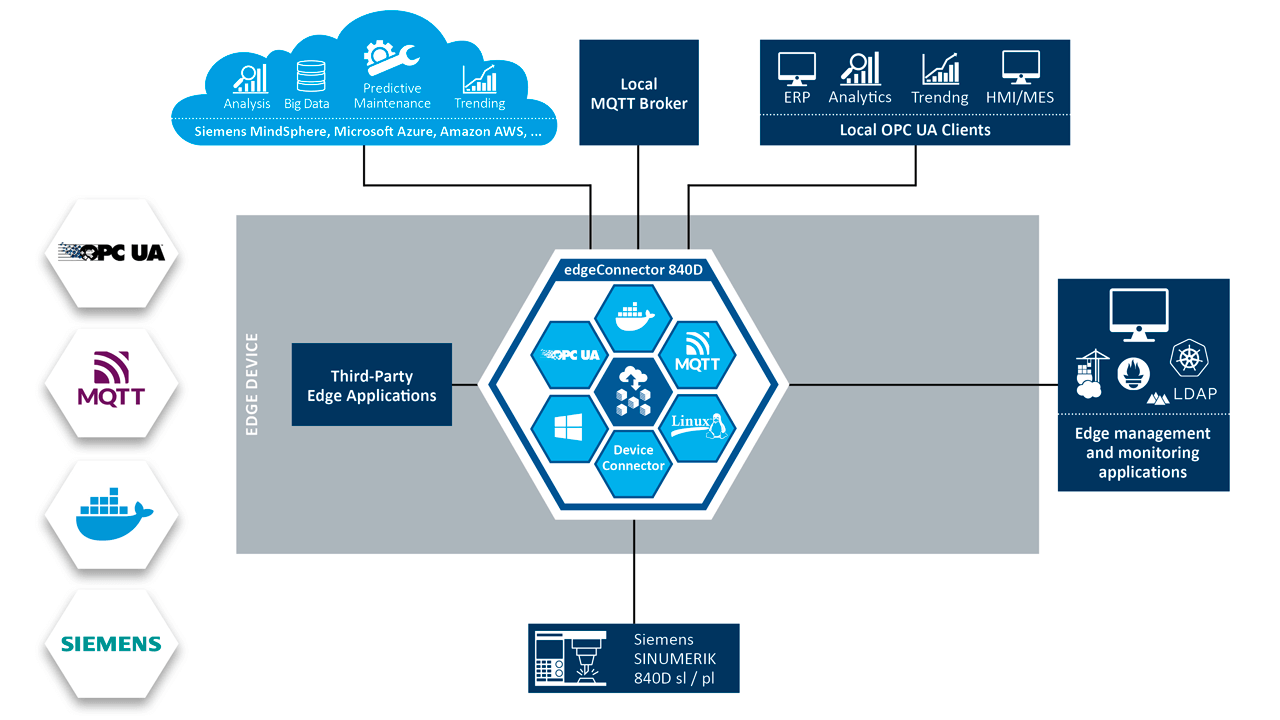
The containerized software modules from Softing Industrial edgeConnector Siemens, edge Connector Modbus, and edgeConnector 840D can be used to implement machine connectivity for many applications.
Software-driven innovations
Standard IT solutions combined with sophisticated software algorithms.
Dr. Christopher Anhalt, Vice President Product Marketing, Softing Industrial Automation GmbH said that “nowadays, innovation in industrial production is software driven. For deeper process integration, more efficient and more flexible production processes, and “smarter” products – software is usually key.”
He said that it may help to distinguish two separate aspects of this situation. First, there are established software technologies, IT standards, proven products and services, which can help to build economies of scale. Cloud technologies fit that description. In some vertical markets, the adoption of cloud started much earlier than in industrial production. Currently, we observe how the industrial market segment is taking more and more advantage of standard IT and cloud, too.
Second, there is software-driven innovation which enables progress and new solutions, specifically for the industrial segment. Sophisticated algorithms for artificial intelligence and machine learning are examples that drive the development of new solutions for industrial production.
“It is the combination of both aspects, the potential of standard IT to build scalable and efficient solutions, plus the potential of sophisticated software algorithms to solve specific challenges in industrial production processes, which drives the increasing adoption of edge- and cloud technologies in the industry,” Anhalt said.
Industrial edge and cloud computing
Anhalt said that, as outlined above, edge- and cloud computing goes hand in hand with economies of scale and the benefits that standard IT technology can bring to any industry. In particular, edge and cloud computing help to cut costs for IT infrastructure and for operation, independent of the specific software application and its benefits.
In this context, it is also worth noting – again not a technical benefit, strictly speaking, but still – that many edge and cloud computing solutions require limited investment. Software can be consumed as a service, with pay-per-use or consumption-based pricing models. This means there should be room for trial and error, as cost can be easily scaled up and down.
“Regarding technical benefits, it is the combination of edge and cloud computing which lets user take full advantage of software and innovative applications. For example, complex, AI based models for machine learning may require the computing power and resources of a central cloud platform. The result on the other hand can be deployed on edge, taking full advantage of reduced latency times,” Anhalt said.
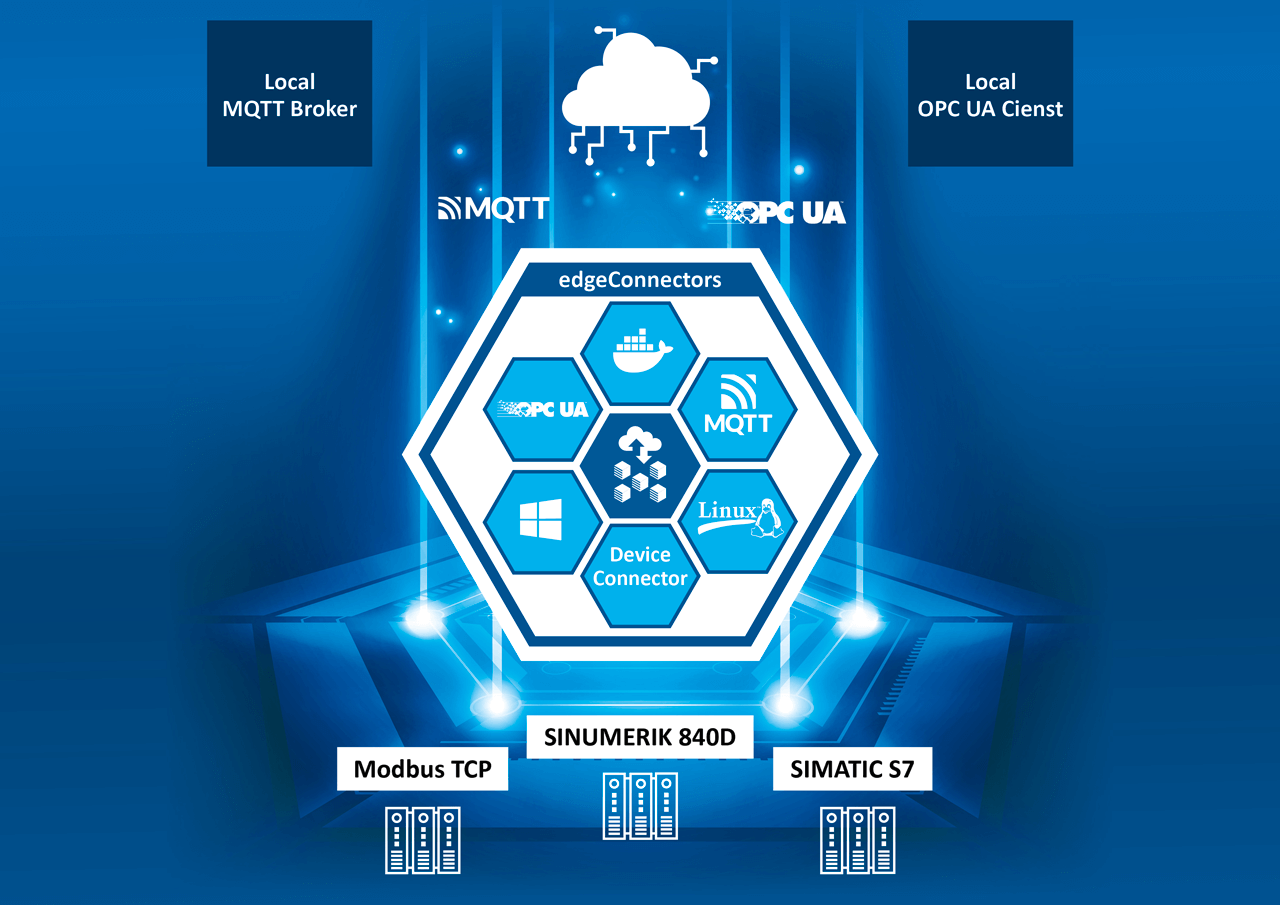
The Docker-based edgeConnector 840D connects SINUMERIK 840D controllers to industrial IoT applications.
Unique technology
When asked to provide a technical description of what makes this technology unique, and how it is being applied to industrial applications, Anhalt cited two examples. First, software virtualization, which is a generic standard IT technology. Second, the enrichment of machine interfaces with semantic information, is a challenge that is more specific to industrial production environments.
“Software virtualization, the availability of software modules as so-called containers, means that software can be deployed largely independently from the hardware layer. The same software module can run on a simple IPC, on a rack server, or even on a central cloud platform,” Anhalt said. “Users can choose between standard software products to manage container-based software solutions. As a specialist in machine connectivity, Softing Industrial offers containerized software modules that can be used to implement machine connectivity for many applications. The software can be managed efficiently at the edge level through IT-driven operational concepts.”
Now, as for semantic information, machine data as it is made available by usual machine- and device interfaces in brownfield projects, is unstructured. With new software solutions for edge and cloud, it is now possible to enrich such unstructured data with semantic information and to provide central governance for such mechanisms.
“Working with an interface that contains semantic information makes it easier for software developers to create their applications. The benefits are multiplied because such a semantic interface can be defined in a unified, abstract way. In this way, multiple machines from different vendors or even multiple production sites look the same for software applications running on a central platform. The OPC UA standard and information models as defined by this standard play a major role in this context. R&D organizations are currently looking at how to apply AI to simplify the creation of information models and to map unstructured data into such models,” Anhalt said.
Industrial edge and cloud solutions
Speaking about edge and cloud applications requiring access to machine data, we have seen many PoCs and trials for quite a few years. But broader rollouts in production have remained slow or have not happened yet at all. So, on the application level in real-world projects, we still see the usual suspects that continue to dominate the discussion, rather than highly sophisticated applications taking full advantage of the latest developments in AI and machine learning.
As might be expected, energy management and related aspects of sustainability are relevant today. Predictive maintenance, tooling, and job scheduling are interesting for every company operating CNC machines. Basic dashboarding and OEE applications, to compare efficiency and performance between multiple production sites, for example, continue to offer benefits for many users.
As outlined above, edge and cloud computing addresses generic questions of IT infrastructure as well as the deployment of the most innovative software algorithms in a broad range of industrial applications. One could argue that there is hardly a challenge that automation engineers face which cannot be addressed by edge and cloud.
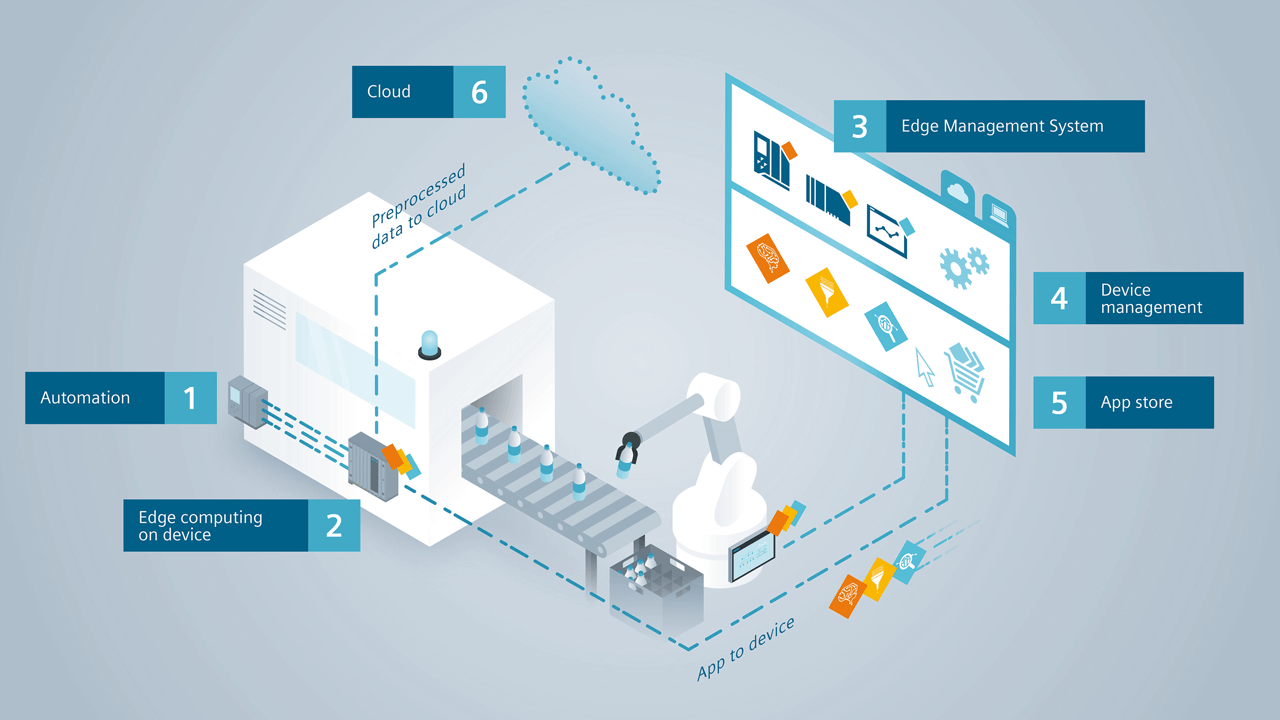
Integrated computing and software environment from automation utilizing edge computing on devices to the cloud using preprocessed data.
System interoperability
Importance of openness and no proprietary technologies, interfaces or protocols.
Marc Fischer, Global Marketing Manager Industrial Edge at Siemens said that, as more devices generate more data, the need for real-time processing, analysis, and actions directly next to the production or machine control becomes even more critical, making edge computing an attractive solution.
“Artificial intelligence and Machine Learning have been integral in providing the processing capabilities required to analyze the large volumes of data generated by IoT devices. These technologies provide benefits at production level / shop floor or even in the cloud. Low coding and ready-to-use frameworks and tools now also enable experts from the “traditional” automation world to use these technologies and thus generate a value add,” Fischer said.
“Edge computing and cloud computing are the infrastructure to deploy and manage such applications. Moreover, virtualization is increasingly reaching the shop floor because software can be hosted much more flexibly, the administrative effort is reduced because much is centralized, and the computing power of virtualized and containerized infrastructures can be dynamically allocated and always assigned to those processes that currently need it.”
He added that cybersecurity is becoming increasingly important and computing power is becoming cheaper and can also be used on the shop floor for very compute-intensive processes. This also enables industries with very high IT security standards and sensitive data or critical processes to enable edge computing applications without a cloud connection and to keep all data and processes on-premises.
Potential impact on manufacturing
Fischer said that Industrial Edge brings IT computing power to the shop floor, providing flexibility to keep sensitive data locally or send pre-processed data to a centralized data center or cloud server. This allows manufacturers to have full control over their data.
“It’s particularly beneficial when low latency, high bandwidth, and data security are required. It can also facilitate the convergence of Operational Technology (OT) and Information Technology (IT) by enabling data transparency from the shop floor to the IT level. For instance, Siemens Industrial Edge is used to collect and combine data from different sources, closing the gap between the shop floor and the cloud, and enabling end-to-end vertical data integration,” he said.
Further advantages of Edge Computing with Siemens Industrial Edge: IT (analytics, AI/ML, etc.) is brought to the shop floor in a convenient and standardized way and running directly alongside automation, hence merging the two worlds of PLC programming and high-level language programming or low-coding for IT/OT convergence.
“You can also manage edge applications and associated software and hardware from a central location across machines, lines or even production sites, even worldwide. When productively rolling out these systems (we are talking about hundreds or thousands of edge PCs in case of doubt), significant savings can be achieved through economies of scale,” he added.
Distributed information technology
Edge computing is a distributed information technology architecture that brings data processing, analysis, and intelligence as close as possible to the endpoints that generate and use the data.
The key idea is to process the data at the “edge” of the network, near the source of the data, rather than sending the data to a centralized data processing warehouse. This architecture helps reduce latency, save bandwidth, and provide real-time or near-real-time data analysis and insights.
It also supports decentralization and security by enabling data to be processed and shopd locally, reducing the risk of data breaches and cyberattacks associated with data transmission. It’s being applied in real-time monitoring and data analytics and control of industrial processes, predictive maintenance, and quality control, for example based on AI enabled vision applications. Cloud computing complements this by providing scalability and flexibility, enabling advanced analytics, and AI-powered decision making.
Fischer said that the latest Industrial Edge and Cloud solutions are targeting areas like predictive maintenance, real-time process control, and quality inspection. These solutions leverage IoT to connect devices and systems, creating a more integrated and intelligent manufacturing environment. The result is enhanced operational efficiency and productivity.
“It enables data collection from heterogeneous Shopfloor systems and various automation protocols (S7+, Ethernet/IP, SLMP, Modbus TCP, OPC UA, etc.) including standardization and processing of those – plus: buffering and storage in local data lake and even contextualization with semantic information. Then we talk about connectivity of those shopfloor data sets with upper-level systems such as production or company IT like SAP or MES systems but also cloud / IoT Platforms for further, global analysis. It is only sent to the cloud what’s needed there,” Fischer said.
Another example is analyzation of production data from controller, drives or sensors, etc. to create transparency via dashboards, identify anomalies to predict failures, or to optimize production process and quality of produced goods, e.g., through AI/ML enabled applications.
Finally, there’s a trend for virtualization, also of control systems and to run this combined with other edge computing applications on one single Edge platform and device (maybe also running centrally on a server within the production site).
Addressing challenges
There are several challenges that automation engineers face which edge computing and cloud computing can address. For instance, real-time data processing: automation systems often require rapid responses. Edge computing processes data at the source, significantly reducing latency and enabling faster decision making, which is crucial in many automation tasks.
Data overload: With the rise of IoT devices, automation systems generate large volumes of data. Edge computing can manage this by processing and filtering data at the source, sending only the necessary data to the cloud or central servers, saving bandwidth and storage costs.
Security and privacy concerns: Automation systems often deal with sensitive data. By processing and storing data locally, edge computing enhances data security and privacy, reducing the risk of data breaches and cyberattacks.
Operation technology and information technology are two different worlds: Starting an edge or IoT project, both stakeholder sides within a company need to work together with a clear target from day 1 on. OT experts want to benefit from values of the systems and need to consume the information in a convenient way, whereas the IT colleagues need to buy, install, and maintain software and maybe bring in high language programming expertise.
“There are many hurdles from proof-of-concept to a productive roll-out,” Fischer said. “The overall system must comply with industry standards and components must work together smoothly, also in regard of maintenance. This is solved by ready-to-use and compatible hardware and software, i.e., from Siemens and third party and the possibility to integrate open source or own components into this system. Rolling out updates and configurations for up to thousands of apps and devices worldwide requires central tool and infrastructure for various applications, thus central management functionality.”
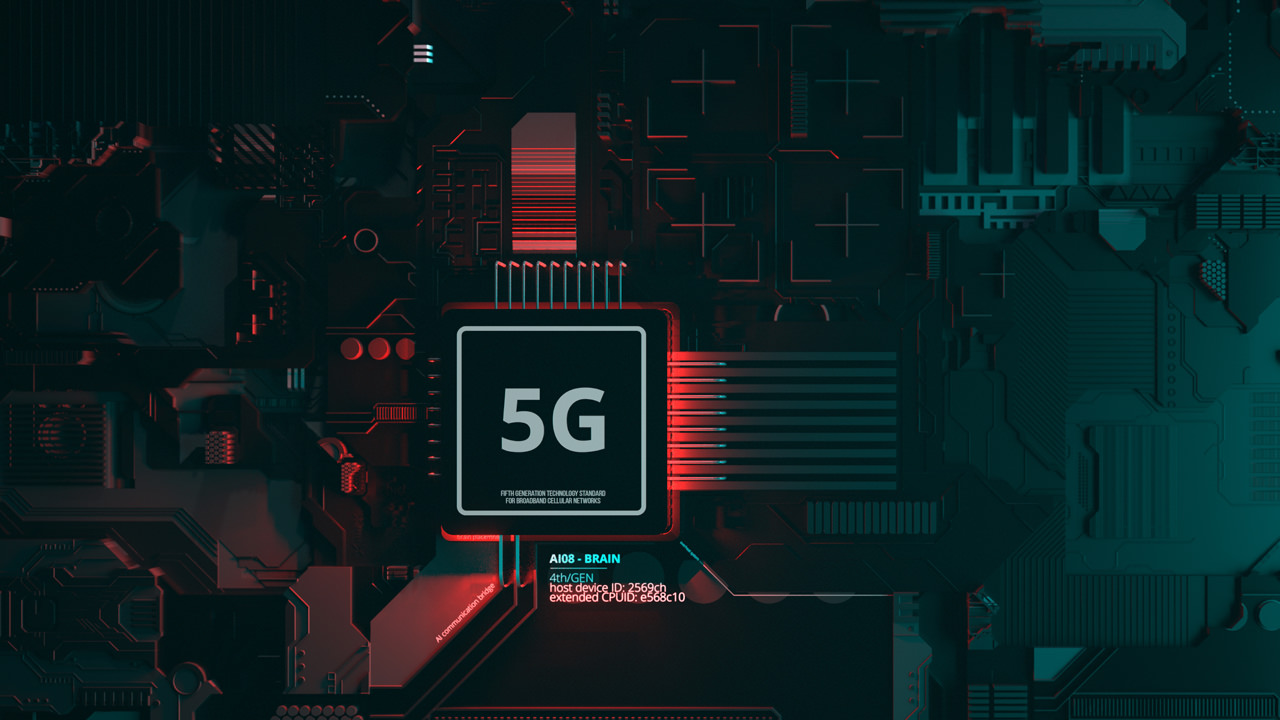
Industrial 5G is another key technology contributing to the development of edge and cloud computing solutions.
System interoperability
Importance of openness and no proprietary technologies, interfaces or protocols.
According to Patricia Yancey Neimes, commercial portfolio manager for software and control at Rockwell Automation, the term ‘Industrial Internet of Things’ (IIoT) has been around for years and is synonymous with the widespread adoption of IoT devices in industrial settings.
With the ever-increasing need for data-driven solutions, IIoT is only accelerating. But it’s not the only thing that’s leading to the emergence of industrial edge and cloud technologies. Here are four more trends that are helping drive more efficient and intelligent industrial operations:
Edge compute: This trend is a companion to IoT devices in that it involves processing data near the source instead of sending it to a centralized data repository. The result? Faster processing, reduced network latency, and improved reliability. For a compute device, this means local control and real-time analytics.
Artificial intelligence (AI): Everywhere you turn these days, AI seems to be a topic of discussion. Although it hasn’t been widely adopted in the industrial space, the possibilities seem endless – particularly in industrial operation and maintenance. Think of using this technology to analyze large amounts of data from edge devices (and even data in the cloud) to deliver insights and make real-time decisions. What about using that same data in a different way for predictive maintenance? And these are only 2 use cases! AI is here to stay; and we will continue to discover more ways it can be used to deliver optimized, intelligent operations.
Digital twins: Although digital twins aren’t necessarily a new concept, they are increasingly becoming part of the design lifecycle when it comes to industrial automation. As a digital replica of an asset or system, a digital twin provides a virtual model that can test, monitor, troubleshoot, and optimize your process even before the first bolt is ordered. Digital twins also enable concurrent workflows in the design lifecycle that ensure a solid design and support virtual training, whether at the edge or in the cloud.
Cybersecurity: Whether it’s your personal data or data from an industrial system, security is a topic that’s always top of mind. Connection and data exchange is at an all-time high and continues to grow. With that comes an ever-increasing need for resilient security measures. Cybersecurity is an evolving technology that’s critical for protecting data, network infrastructures, and assets from very real cyber threats that continue to attempt to undermine the integrity, privacy, and availability of industrial operations.
She said these are only a few of the trends that are driving the rise of industrial edge and cloud technologies. Some more to think about include: the ever-increasing remote workforce, continued convergence of IT/OT, advanced analytics, robotics, and machine learning.
Potential impact on manufacturing
Kevin Olikara, consulting manager at Kalypso, a Rockwell Automation business, said that “cloud computing provides incredible scalability for data storage and compute resources, in addition to improved redundancy and resiliency. Manufacturing organizations can use cloud computing to collaboratively design and simulate complex industrial systems. In addition, these characteristics make cloud environments ideal as an industrial data hub to manage very large quantities of data, as well as a large variety of data types, including time-series data, events, and engineering data.”
“This helps manufacturing organizations get more value from the vast amounts of data they generate across many disciplines. With an industrial data hub as a foundation, cloud computing is ideal for building analytics applications used across the enterprise, as well as training AI models using those large data sets.”
Olikara said that edge computing provides much lower latency and connection resiliency compared to data being sent to and from the cloud. This is critical when deploying AI applications that process large quantities of data while also running synchronously with manufacturing systems and processes. Key examples of AI applications that need to run at the edge include advanced process control, automated vision inspection, and equipment failure prediction. When deployed successfully, these use cases provide step change improvements in productivity and quality to manufacturers.
“Industrial edge computing itself has been around for decades,” Olikara added. “What’s novel with the latest wave of technology is the convergence of IT and OT, resulting in edge to cloud architectures. Edge to cloud architectures leverage the best capabilities of both ends to enable highly integrated, closed-loop workflows. Ultimately, this enables manufacturing organizations to leverage new IT capabilities while still meeting industrial specific requirements surrounding agility, reliability, and security.”
He said that the data flow starts with deploying data extractors and gateways, like FactoryTalk Edge Gateway, to the edge to ingest data from a very heterogeneous environment that employs many vendors and protocols. Then, a cloud-based industrial data hub, like FactoryTalk DataMosaix, can ingest and contextualize this data from previously disparate sources, providing easy but controlled access to this data by people and applications across the organization.
This data can be used by data scientists to build predictive and prescriptive machine learning models. Leveraging MLOps processes and systems, data scientists iterate and improve these models as more data is collected over time and manufacturing systems change. Edge applications can utilize machine learning models, which can then be deployed across many edge devices using an edge management and orchestration solution like FactoryTalk Edge Manager.
IoT and enterprise connectivity
Adam Gregory, software commercial leader at Rockwell Automation, said that Rockwell has been thinking about how to get the right tools into the hands of the right people based on what they need from an industrial automation platform.
“Over the last year, we’ve talked a lot about the FactoryTalk Design Hub, which is being built for system design, development, and deployment,” Gregory said. “Our main goals here are to enable collaboration across multiple users and skillsets, as well as on-demand access to compute-intensive applications like digital twins that provide controller emulation and system simulation. All of this is enabled by our use of the cloud, providing a single point of access for all collaborators, and the scalability of exactly the number of resources you need, when you need them.”
He added that, for people who are working directly with production systems, either creating product as operation engineers or keeping them running as maintenance engineers, we’re providing cloud solutions for production performance management with Plex and CMMS workflows with Fiix.
All these workflows benefit from the industrial edge, whether you’re connecting from the cloud to a device to deliver part of your project or gathering data from the production system to aggregate and communicate out to the cloud for advanced analytics. We recognize that the collection of production data, as well as safe and secure communication into and out of a production network is critical no matter how you fit into the industrial automation system.
Engineering challenges
Patricia Yancey Neimes said automation engineers are faced with more challenges than ever before. As automation continues to be adopted to improve productivity, reduce costs, and increase efficiency, engineers must navigate a complicated landscape of interoperability issues, complex infrastructures, and the ever-increasing pace of technological advancements.
Keeping abreast of these advancements while ensuring the security of operations is daunting enough, not to mention the ongoing pressure of delivering innovative products to customers. She added that are some other challenges facing today’s automation engineers and how industrial edge and/or cloud solutions may help:
Data silos: Most automation systems are a constant work in progress. Ever-changing customer demands require new production workflows, which means looking for new ways to work smarter and faster. Sometimes that means adding new solutions to industrial operations which can introduce yet another hardware/software interface and data silo into the mix.
Heterogenous data environments with lots of different data types and sources are a reality in most production systems. The trick is to find a way to use the data at scale across many applications. Cloud-based solutions like FactoryTalk DataMosaix helps with this by providing simple access for complex data to automation engineers. This industrial DataOps solution takes data from different sources, contextualizes it, and delivers it to a range of applications across the organization.
Scalability/flexibility/agility: The need to respond to ever-changing requirements in industrial operations means this topic will continue to be something automation engineers will face for the foreseeable future. Ensuring these attributes throughout the operate and maintain lifecycle can be daunting, especially at the speed that business is happening today. The rise of edge devices has created unique challenges and complexities to manage these devices at scale. OT-focused edge management tools like FactoryTalk Edge Manager help automation engineers more efficiently manage edge devices and applications.
“In the design space, cloud-based tools make design teams scalable, agile, and flexible by allowing anytime, anywhere access via a web browser without the need to download, install, or activate software (think FactoryTalk Design Studio),” she added. “Additionally, simulation software, modeling applications and analysis/optimization tools can be accessed to make the design phase more agile and decrease time-to-market (think FactoryTalk Twin Studio).”
Latency: Industrial automation systems often require real-time decision-making. Bringing computing resources closer to the edge minimizes the latency associated with sending data to a remote cloud or data center for processing. This reduced latency enables faster responsiveness and improves the overall performance of time-sensitive automation applications.
Cost: When trying to find solutions to challenges in the workplace, it always seems to come down to cost. When it comes the bottom line, industrial edge and cloud deliver distinct value. Concerned about the potential capital expense of a cloud infrastructure and the related data transfer costs? Industrial edge delivers cost savings by processing and storing data locally. How? Through resource optimization and reduction in cloud services.
The cloud has its own set of related cost efficiencies. One example that comes to mind is that of creating a digital twin for a control system. Software that creates system models can be cost prohibitive – especially when one or both may only be used during the design process. Instead of making that investment, engineers can take advantage of cloud-based solutions like FactoryTalk Twin Studio that use a pay-as-you-go pricing model for compute power, storage, and services, avoiding capital expenses in favor of operational expenses.
Collaboration: Engineering teams are working remotely now more than ever. The rise of cloud technology has been key to making geographically dispersed teams a reality, supporting the ability to access shared resources and work simultaneously on the same project while using integrated version control to protect the integrity of the project.
“The challenges facing automation engineers today seem endless, but new and innovative edge and cloud solutions are making those challenges more manageable,” she added.