TechnologyJuly 23, 2024
Industrial Edge Computing Megatrends: Special Report
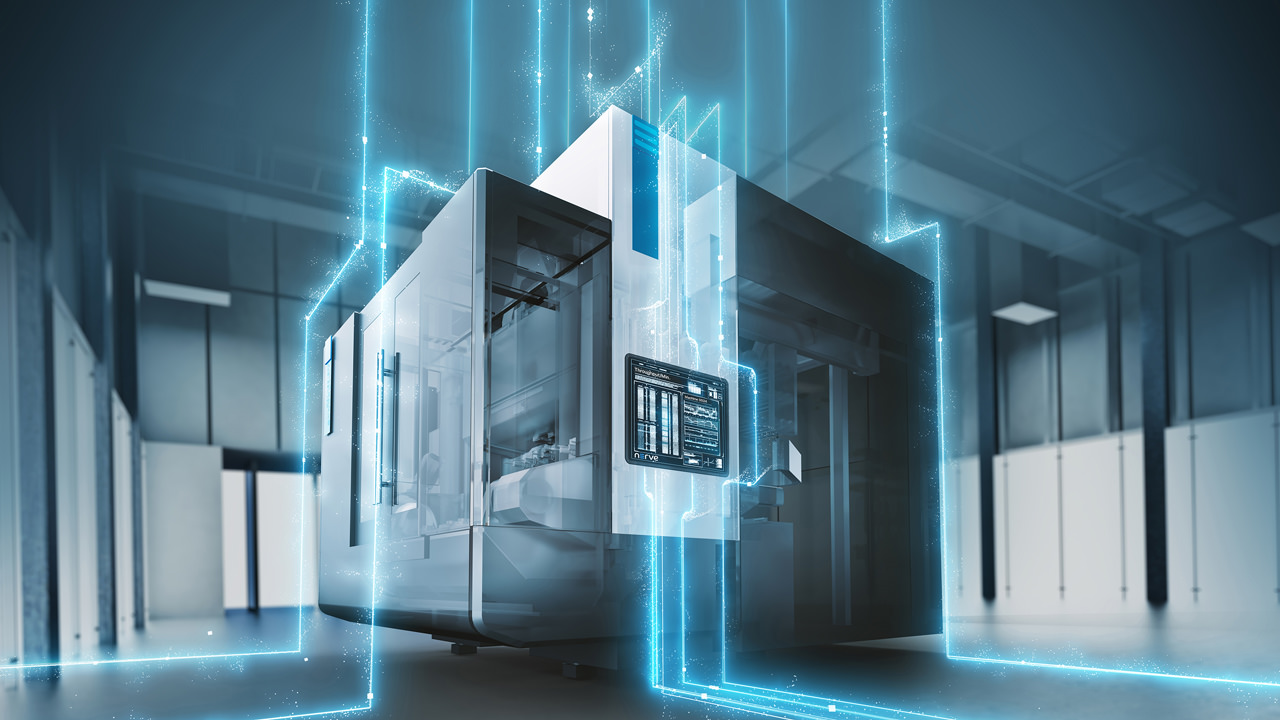
Industrial Edge and Cloud Computing are among the most exciting areas of technology development for smart manufacturing. This Industrial Ethernet Book special report provides the perspective of nine industry experts as they share their shared vision for the future of the Industrial Edge and the megatrends that are pushing initiatives forward.
Industrial edge and cloud computing has a bright future. With all the latest advancements in artificial intelligence, cloud computing and data management, the next generation of industry will be more secure and intelligent than ever before.
What makes edge-computing unique and different is how networking devices are now being mobilized as compute nodes, replacing dedicated hardware resources that were commonly implemented for the purpose in industrial networks.
For this update on the Industrial Edge, we reached out to a series of industry experts to get their perspective on the technologies that are shaping the present and future developments with the Industrial Edge. Each provides a unique view of its potential impact on smart manufacturing, and how companies can leverage this unique ability to bring data processing closer to the plant floor.
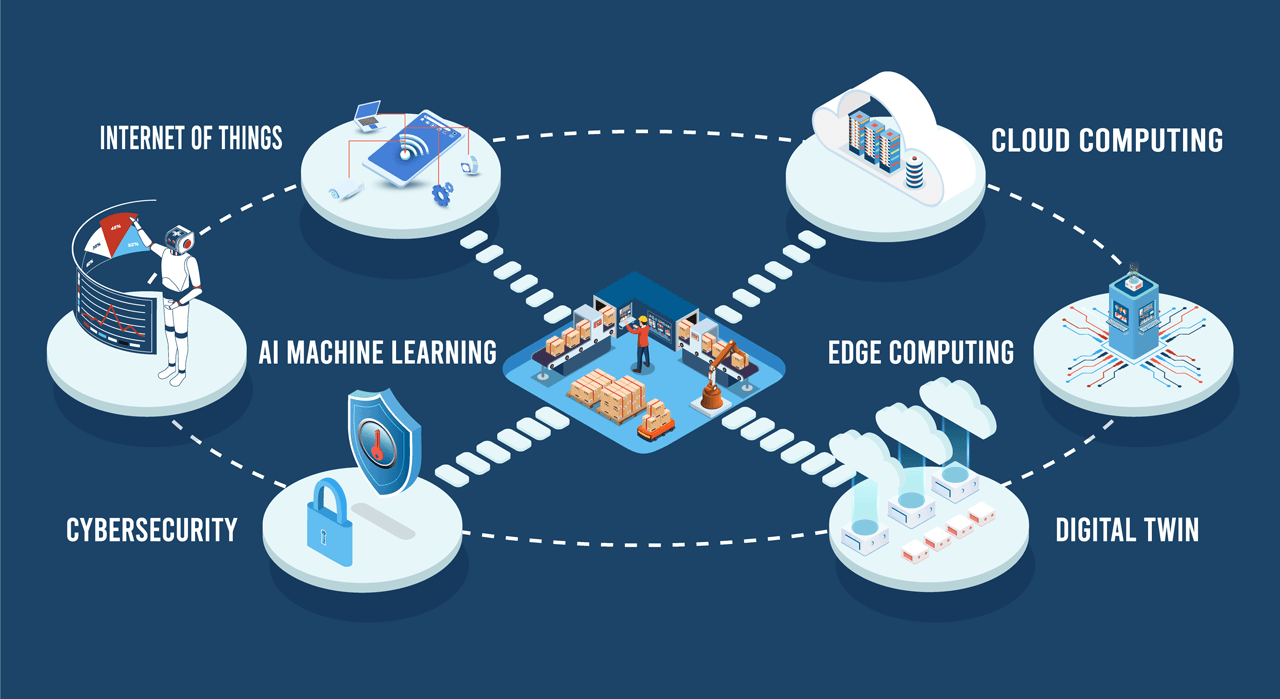
“Edge computing plays a crucial role for localized data processing with reduced latency and improved security and privacy. Software technologies driving these advancements include containerization with Docker and orchestration with Kubernetes, and edge AI frameworks that can work with resource constrained hardware platforms,” Georg Stöger, Director Training and Consulting at TTTech Industrial.
Real-time data generation and preprocessing
Localized data processing with reduced latency and improved security and privacy.
“Technology trends that make more complex and powerful Industrial Edge and IIoT solutions possible include widespread sensor deployment for real-time data generation and data preprocessing at the edge; wired and wireless high-speed, low-latency networks for interconnecting more I/O and processing units locally at the edge; and AI for predictive maintenance and enhanced decision-making,” said Georg Stöger, Director Training and Consulting at TTTech Industrial.
“Edge computing plays a crucial role for localized data processing with reduced latency and improved security and privacy. Software technologies driving these advancements include containerization with Docker and orchestration with Kubernetes, and edge AI frameworks that can work with resource constrained hardware platforms. Federated learning has come up more than once as an AI technology trend; this technology can address resource constraints, privacy concerns, and scalability in edge-centric applications of various verticals including industrial automation,” Stöger said.
Stöger added that cybersecurity remains an overarching key topic for edge, cloud, and IIoT systems, especially in the EU, where the NIS2 directive is going to take full effect by the fourth quarter of 2024.
Why it matters
Stöger said that edge computing brings data processing closer to the source, reducing latency and bandwidth usage, which is essential for real-time decision-making and rapid response to operational changes. Localized processing improves reliability and security by minimizing the transmission of sensitive data over networks.
Cloud computing provides scalable storage and computational power, allowing manufacturers to handle large volumes of data and complex analytics without hardware constraints. Also, cloud computing can perform analyses and optimizations based on a global view of process data from multiple assets, which is not available at each edge device.
“The combination of edge and cloud computing enables seamless integration of IoT device data collection, advanced analytics, and AI/ML models, leading to a more connected and intelligent manufacturing environment,” Stöger said.
By leveraging these technologies appropriately, manufacturers can achieve higher levels of automation and operational efficiency. The integration of industrial edge and cloud computing transforms manufacturing into a more agile, data-driven, and intelligent industry, paving the way for innovations and more sustainable manufacturing practices.
Uniqueness of technology solutions
According to Stöger, industrial edge and cloud computing bridge the gap between IT and OT infrastructure; they integrate real-time data processing and advanced analytics with control infrastructure that used to operate in isolation due to technical constraints and security concerns. Unlike past solutions that relied heavily on centralized computing, a hybrid edge/cloud infrastructure can utilize containerization technologies like Docker and Kubernetes which orchestrate and distribute computational tasks across the edge and the cloud.
Edge devices often provide substantial computational resources, allowing them to perform data acquisition, data (pre)processing, AI inference, and decision-making locally on a single device, possibly combined with local HMI functions. This results in significantly reduced processing latency and more cost- effective infrastructure. The use of and orchestration tools such as Kubernetes facilitates the development, deployment, and management of applications across diverse environments, ensuring consistency and scalability.
Past implementations often struggled with scalability and flexibility due to hardware limitations and rigid infrastructure. The new approach leverages the cloud for its storage and processing power. The integration of robust cybersecurity measures also marks a significant improvement, as contemporary solutions should be designed based on a comprehensive cybersecurity framework which systematically protects distributed networks and data flows.
Focus on innovation
“Machine builders and energy management systems are among the industries which can benefit substantially from these solutions,” Stöger said.
“In industrial automation, edge/cloud computing can create highly responsive and adaptable production lines. Real-time monitoring and control enabled by edge computing allow for immediate diagnostics and adjustments of machinery, enhancing productivity and quality and reducing downtime. For machine builders, this means the ability to offer more sophisticated and reliable equipment, equipped with predictive maintenance capabilities that anticipate failures before they occur,” he said.
Stöger added that energy management for industrial production systems benefits from real-time data analytics that optimize energy consumption and distribution, improving efficiency and reducing costs. These systems can help to predict and even dynamically adjust energy flows in response to demand fluctuations, leading to more sustainable and efficient operations.
Advances in artificial intelligence, particularly federated learning, are playing a crucial role in these developments. Federated learning allows AI models to be trained across multiple decentralized devices or servers holding local data samples, without exchanging the data itself. This is particularly beneficial for systems where sensitive data privacy and security are paramount. By deploying federated learning, system builders can develop and refine AI models directly on the edge devices, ensuring that the models are continuously learning and improving from local data while maintaining data privacy. This decentralized approach not only enhances data security but also reduces the latency and bandwidth required for continuous data transfer to the cloud.
Looking to the future
“As edge computing continues to evolve, it will incorporate more advanced AI and machine learning applications directly at the point of data generation, enabling real-time analytics and decision-making capabilities. This localized processing will reduce latency, increase reliability, and enhance data privacy, driving more efficient and responsive industrial systems,” Stöger said.
“Looking at one technology in particular, we believe the integration of federated learning will allow for continuous improvement of AI models while maintaining data security, facilitating smarter and more adaptive industrial processes. As these technologies become more widely available and their potential is better understood, they will drive further innovation in predictive maintenance, energy management, and automated quality control, leading to an even more interconnected, intelligent, and efficient industrial landscape,” Stöger said.
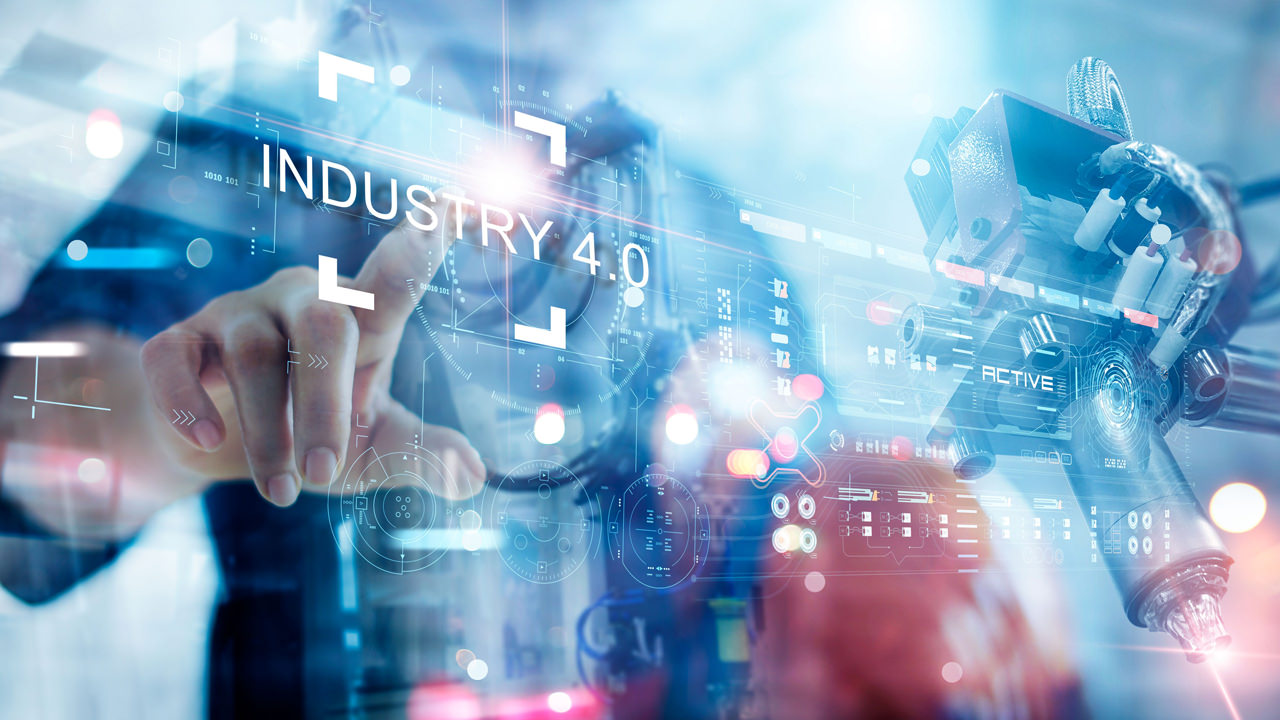
” It’s the security features on today’s edge devices that enable secure data transfer to the cloud, which in turn enables advanced cloud computing. Publish/subscribe communication technologies like MQTT Sparkplug, a new international standard, publish data only when changes occur, reducing unnecessary data flow and network traffic,” Dan White, Director of Technical Marketing at Opto 22.
Industrial Edge megatrends
Focus on security and flexible choices among programming platforms.
Dan White, Director of Technical Marketing at Opto 22 said that “it’s the security features on today’s edge devices that enable secure data transfer to the cloud, which in turn enables advanced cloud computing. Publish/subscribe communication technologies like MQTT Sparkplug, a new international standard, publish data only when changes occur, reducing unnecessary data flow and network traffic. Support for SSL/TLS certificates ensures that those connections are securely authenticated and encrypted. Outbound connections from your edge devices means no inbound ports are open—significantly reducing cyber attack vectors. IT departments can enjoy user authentication that supports lightweight access directory protocol (LDAP), the same tools used on every PC in your plant.“
Flexible programming platforms
White said that flexible programming platforms on edge devices are another key cloud enabler. With open-source friendly software tools, developers can work with languages and protocols they prefer. Tools like user defined data types (UDTs) can add context to raw data, so when it arrives in the cloud, it already has meaning and context—rather than clumps of raw data that muddy the waters.
On the cloud, software technologies like artificial intelligence and machine learning, which require vast amounts of storage and processing capability, enable data scientists to perform powerful analysis to improve future operations. Using commonly understood programming tools like structured query language (SQL), cloud computing makes it easy to work with enormous data tables. This simply can’t be done at the edge with limited computing resources,” White said.
White added that edge technology is the lifeblood of operational technology. After all, you can’t rely exclusively on cloud platforms like AWS and Snowflake to run a machine on the plant floor—it’s just not practical. A simple network outage would cripple your operations. Neither can you rely on edge computing devices to analyze large swaths of data to gain better insight into your operations—edge devices aren’t built with that kind of computing power.
Design your edge and cloud architectures with each other in mind. Choose edge devices that support the technologies that the cloud relies upon. Ethernet protocols that are standard, like REST APIs, MQTT Sparkplug, OPC UA, and even legacy protocols like Modbus and Ethernet/IP all play important roles in the technology stack from the plant floor to the cloud,” White said.
He added that the impact of a cohesive edge-to-cloud architecture is substantial and used a car analogy. Edge devices are new modern EVs that are sending all their data to the cloud to improve self-driving and safety algorithms. The algorithms are regenerated periodically with the best and most current data, then they get sent down to the vehicle in the form of a firmware update. After each update, the vehicle operates more safely and drives better than ever before. The EV never relied upon the cloud for real time driving control, but the better driving algorithm would not be possible without cloud computing power to process data from millions of cars worldwide. Not to mention the global visibility—the car manufacturer can anticipate potential safety, maintenance, or service issues before the driver.
Unique technology solutions
White said that “the newest technology is universal, not proprietary” For example, a PLC with its own REST API would have been a completely foreign concept just a few years ago, but now there are a number of them available on the market. In fact, some PLCs now come equipped with REST APIs, OPC UA servers, and MQTT clients/servers—along with legacy protocol support like Modbus, ProfiNET, and Ethernet/IP.
Engineers and designers are tired of being locked into closed, proprietary systems versus a system that uses an architecture built on open standards. In the past, most edge devices were part of closed systems—like a PLC with a dedicated HMI and a proprietary Ethernet protocol for communications. What was once the status quo has quickly become an antiquated architecture,” White said.
And don’t confuse open standards and open source friendly communications with vulnerability. We’re talking about the same types of communication and security methodologies that people use every day for online banking, private healthcare data, and sensitive corporate communications,” he added.
As management demands that more operational data be available, legacy proprietary systems have proven to be a bottleneck for valuable plant floor data. In contrast, IT professionals who are looking to get data from modern edge devices have no trouble understanding the standard communications onboard.
Applications focus
OEM machine builders and service companies have a lot to gain from the latest edge and cloud solutions. In the past, machine builders would deploy their machine to a customer site and walk away. Typically they’d get a phone call or email for service when the machine failed. The service model was purely reactive. It often left the customer with costly downtime situations and hurt the reputation of the machine builder.
Machine builders who are taking advantage of modern edge-to-cloud architectures can benefit immensely. Machines deployed in the field are continuously providing key data—and with cloud computing power—machine learning and AI algorithms can improve designs for the machine of the future.
And even more importantly, OEMs can monitor their machines in real time and get alerts, often learning of a potential failure or downtime situation before the customer. With this kind of knowledge, the OEM can provide better service at a lower cost than ever before—a win-win scenario.
Looking ahead
Software containerization is emerging as a new tool on industrial edge solutions. Software containerization is a method of packaging an application and its dependencies together so it can run reliably on any computing environment. Containers make custom software deployment on edge devices simple and consistent,” White said.
By reducing conflicts and dependencies, software containerization enables rapid, scalable updates. Containers can be added or removed quickly based on current needs, allowing flexible resource management, which is crucial for handling varying workloads on edge devices. Plus, containers are lightweight, optimizing the limited computing resources available on edge devices.
With software containers, you can apply new versions or fixes quickly without significant downtime, ensuring that edge devices remain up-to-date and efficient. This adaptability and efficiency are vital for advancing IIoT and Industry 4.0,” White concluded.
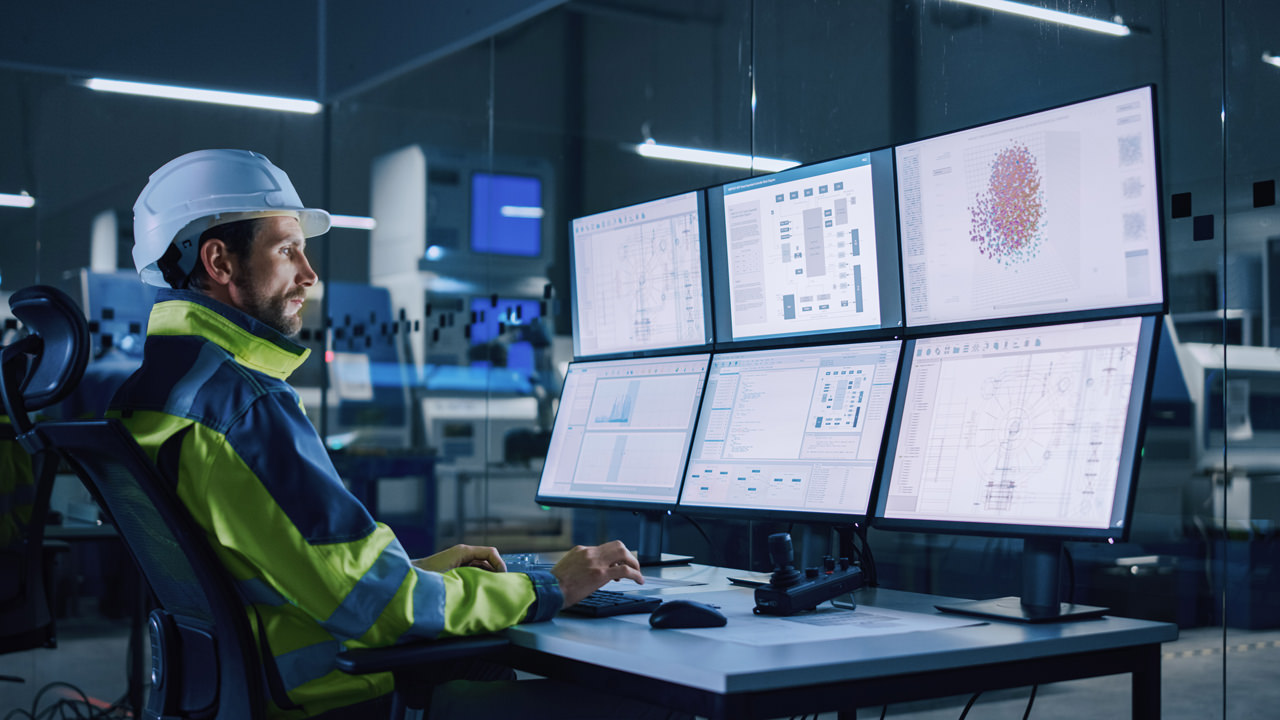
“Industrial manufacturing is highly programmed and full of automation, but the future of smart manufacturing requires more autonomy. Building autonomous systems for manufacturing will require new datasets of clean and trusted data, machine learning applied on top of first principles, and ways to manage scaled deployment to distributed automation systems,” Oliver Haya, business manager and Adam Gregory, software platform manager at Rockwell Automation.
Emergence of Containers and Clusters
Unlocking new value propositions for security, collaboration, and scalability.
According to Oliver Haya, business manager, and Adam Gregory, software platform manager at Rockwell Automation technology megatrends are enabling new levels of Industrial Edge and Cloud Computing solutions.
Cloud computing has been growing steadily for years across every industrial vertical. However, the use cases were limited by the costs of applications in the cloud, the challenges of large-scale data from manufacturing, and network security through the plant operations. Many applications that moved to the cloud started as a lift-and-shift of existing on-premises software, significantly limiting the cost benefits and functional value of what the cloud can offer.,” Haya and Gregory told the Industrial Ethernet Book recently.
New applications are taking advantage of containerization and clusters, making them more portable across cloud and edge and easier to scale. These new solutions also become more affordable with cloud-native and edge-native designs, and unlock new value propositions for security, collaboration, and scalability. As modern security models proliferate into the industrial market, it will become easier not only to bring data from edge to cloud, but also to send data, applications, and AI models from cloud to edge.”
Benefits of new technology
Haya and Gregory went on to discuss the benefits of this technology and how these megatrends are impacting smart manufacturing.
Today, industrial manufacturing is highly programmed and full of automation, but the future of smart manufacturing requires more autonomy. Building autonomous systems for manufacturing will require new datasets of clean and trusted data, machine learning applied on top of first principles, and ways to manage scaled deployment to distributed automation systems. Data contextualization at the manufacturing edge enables better datasets that can be made more meaningful by merging with engineering data from the cloud.
As the data is used to make better design decisions, new code and models need to deploy securely back to the edge for low latency execution. Who’s going to do this? Subject matter experts today are already overloaded, and new engineers often take time to onboard into traditional automation programming tools. Using SaaS can reduce their non-value-added time with automatic software management, seamless onboarding, and GenAI copilots to simplify their life and give them room to innovate toward autonomous operations.
Breaking from the past
“Designing for edge-native and cloud-native applications optimizes in many ways. One of the best aspects of containerized microservices and compute clusters is the ability to scale usage quickly. This makes it easier to get new users and sites running, while also making the adoption more elastic – downsize as you need. Having access across the right users makes it easier to have features that emphasize human collaboration, like SaaS for automation system design that integrates git-based version control and CI/CD pipelines,” Haya and Gregory said.
“The evolution of security to support multi-user and multi-device systems, like zero-trust architectures will ensure only the right actors can engage with the control systems and data systems. As the data systems evolve, new AI paradigms will revolutionize access to data for decision making. Putting all of these together is essential to centrally managing distributed automation systems.”
Applications and industries
Haya and Gregory said that the first prerequisite to benefiting from edge and cloud solutions is a robust network architecture – without standard IT/OT security measures and enough network bandwidth, the value of edge and cloud may not be fully realized. Across industries, companies that have geographically separated facilities can benefit from cloud, as can small companies without extensive IT support staff.
AI-enabled tools that are designed to run at the edge for better latency and lower cost of compute will enable better prescriptive maintenance and improved process control. Mixing edge and cloud will have benefits for reducing carbon intensity per product and controlling energy costs by optimizing production plans with real time data from equipment, utilities, and weather. Connecting data from the edge with cloud-based digital twins will enable better decision making. Along with digital twins, generative AI co-pilots, enabled by SaaS in the cloud, will have widespread adoption throughout the automation lifecycle.
Future of industrial edge solutions
“While automation has been traditionally comfortable with on-premises computing and enterprise data centers, the emergence of edge and cloud are blurring the lines between these – this will continue to create more hybrid deployments,” Haya and Gregory said.
“The fluidity of applications, to be managed from a centralized interface in the cloud while being deployed across many sites, creates a future of software-defined architectures. This will expand the use cases that span edge and cloud to include more design software, more HMI, more MES, more analytics, and more AI/ML deployments. Coordinating versions of PLC code, HMI projects, recipes, and machine learning models from every site into a global corporate discipline will reinforce the learnings and share best practices more easily. Critical to this expansion will be standards-based development, including tools like OPC UA companion specifications and the newly announced Margo Initiative for edge interoperability.”
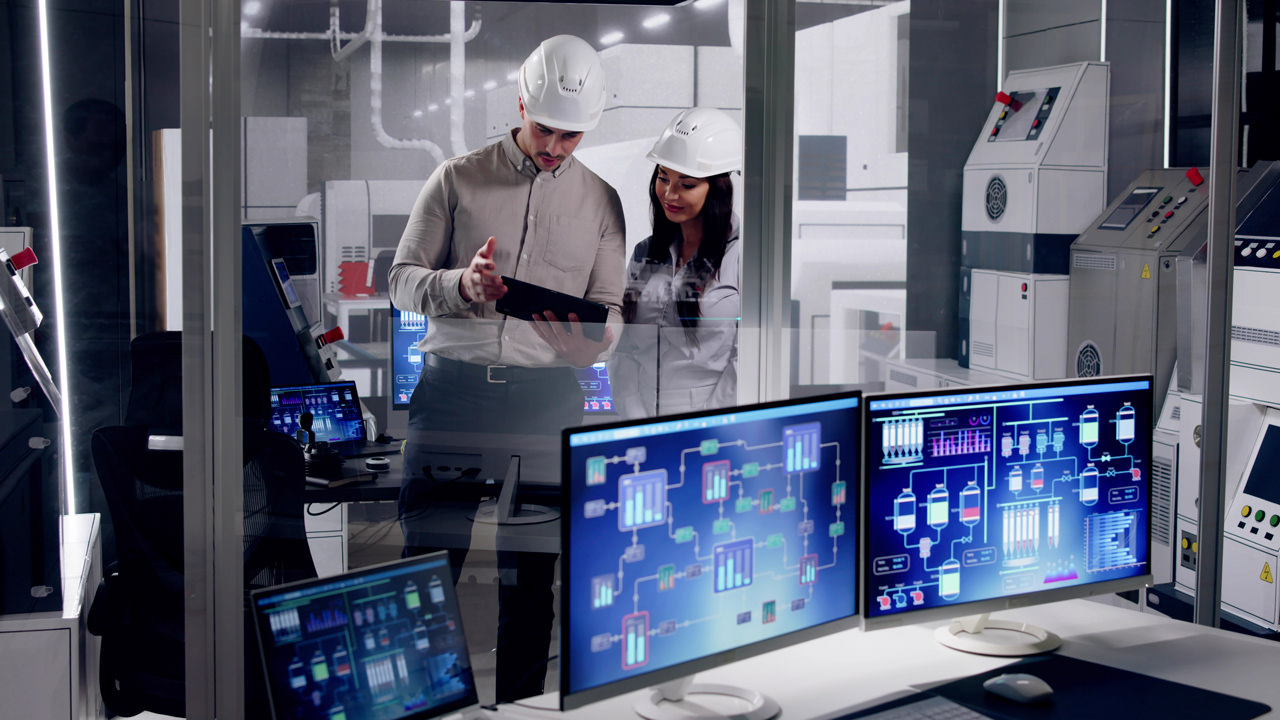
“Wringing the most possible value out of data is becoming crucial if a company wants to be leading edge. The largest companies in the world were mere startups 25 years ago. Harnessing data propelled their astronomical growth. Data and extracting maximum value from it are now essential for nearly all companies,” Dave Eifert, Senior Business Development Manager – IIoT, Phoenix Contact USA.
More power at the Edge
Harnessing data can provide impetus and opportunities for growth.
According to Dave Eifert, Senior Business Development Manager – IIoT, Phoenix Contact USA, “more compute power (whether GPUs or enhanced CPUs) provides much more power at the edge. AI models, in some cases, can be trained at the edge, and in most OT-related use cases, can be run at the edge. AI software that is designed for the Edge is changing the paradigm, allowing ML and AI to avoid the latency and cost of making a round trip to an offsite cloud. The nearly limitless power of the Cloud can be harnessed for particularly demanding AI and ML model training. It also can be leveraged for multi-tenant applications or to serve an enterprise that is geographically dispersed.”
“Wringing the most possible value out of data is becoming crucial if a company wants to be leading edge. The largest companies in the world were mere startups 25 years ago. Harnessing data propelled their astronomical growth. Data and extracting maximum value from it are now essential for nearly all companies,” Eifert added.
He added that, from a technical standpoint, Edge and Cloud computing can be thought of as a way to flatten the communications architecture to a publish-subscribe topology. Data can then be normalized and shared with appropriate applications and people. For example, energy data can be captured and can be synced with production data. It is easy to then see when energy is still being used (wasted) or when production isn’t running.
Very importantly, all this same data can then be used to train ML models that can then be run to find anomalies that the human eye cannot detect. This information can lead to effective preventive maintenance and avoid downtime.
Technology innovations
Eifert said that today’s Edge and cloud-based systems start with the premise that the data is as important as simply controlling the process. The biggest difference is a streamlining of how to get data from the process to all the places it needs to go to help the operation (business) fully flourish.
“From a technical standpoint, that means going from the hierarchical automation pyramid where the bottom layer is comprised of “dumb” sensors and actuators, up to the PLC level where control occurs. The next layer up is the supervisory (or SCADA) level,” Eifert said.
Above this is the Manufacturing Execution System level, and finally the top of the pyramid holds the Enterprise Resource Planning system. In this architecture it is difficult to get data from layer to layer, and to move it horizontally across the layers. The new topology – Publisher-Subscriber allows data producing devices to publish data to a central software hub called a broker. Other devices and applications can subscribe to the broker, creating two-way communications. In this way, the cyber-secure, free flow of data can be established.
Prime applications/industries
Eifert said that all industries are ripe for modernization to digitalization including Edge and cloud solutions. As he stated earlier, data’s effective use has tremendous potential value. Not harnessing it is like throwing away valuable currency. This is true of harnessing data’s value in real time. Logged data can provide additional usefulness when fed into machine learning models that can provide additional insights that humans cannot identify without the assist given by ML.
Generative AI can be used in powerful ways, for example, to enable a non-programmer to simply ask or command a machine to give some information or perform some action, much as when we interact with our iPhones through Siri,” Eifert said.
The future for industrial edge solutions will also contribute to further developments of the IIoT and Industry 4.0.
Think of the billions of people carrying powerful computers around in their pockets. Most could not program these smart phones, but they can derive benefits through using them in intuitive ways,” Eifert said. “The future of Edge and cloud solutions will similarly allow the operator and executive to interact with the machine, the plant, or the enterprise in similarly intuitive ways. Further, machine-to-machine coordination will become mainstream, eliminating human intercession before acting. We’ll be wise to walk that path slowly, and eliminate risks and unintended consequences, but we will move in this direction.”
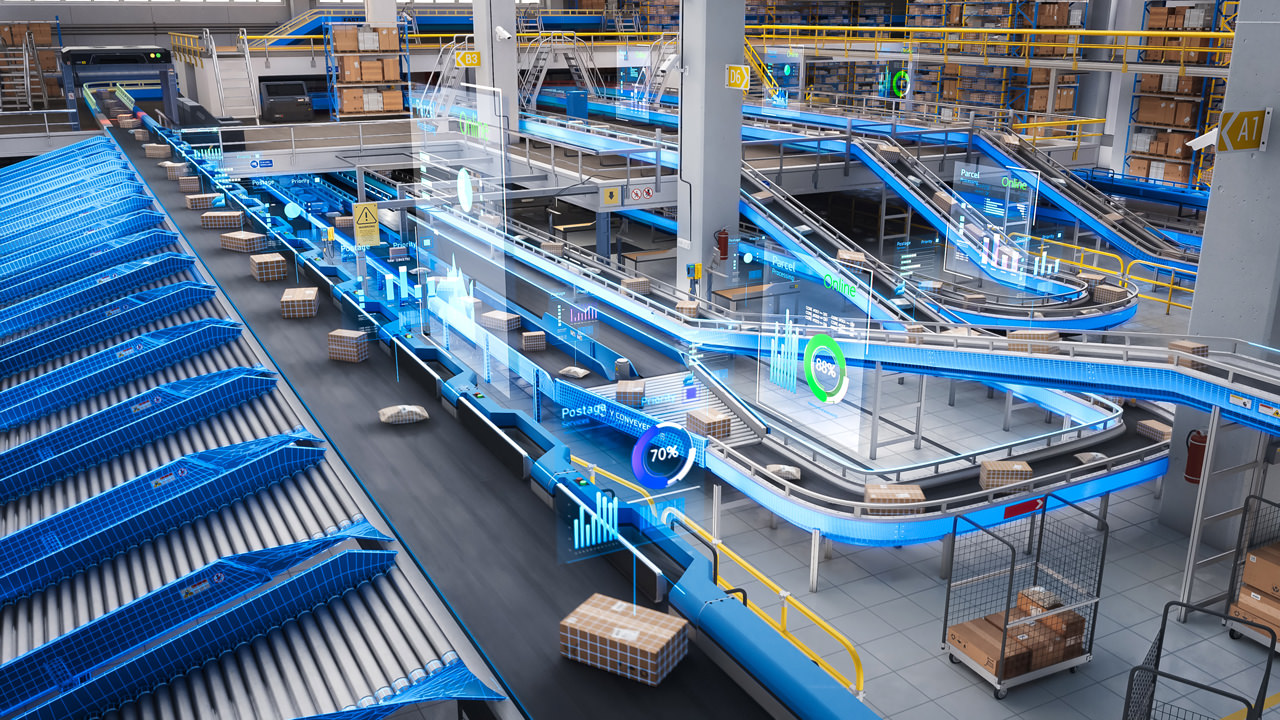
“Industrial edge computing focuses on processing data locally close to the source such as machines, sensors, and control systems. This allows for faster decision-making. Cloud computing on the other hand runs applications located in data centers and is well suited for tasks that require extensive data analysis,” Vivek Bhargava, Product Marketing Manager at Cisco.
Industrial Edge and Cloud
Distinct but complimentary technologies create megatrends by driving innovation.
According to Vivek Bhargava, Product Marketing Manager at Cisco, industrial edge and cloud computing are two distinct but complementary approaches to data processing in industrial environments such as manufacturing plants, or any other location where large amounts of data are generated.
“Industrial edge computing focuses on processing data locally close to the source such as machines, sensors, and control systems. This allows for faster decision-making. Cloud computing on the other hand runs applications located in data centers and is well suited for tasks that require extensive data analysis,” Bhargava said.
“As industries look to adopt new technologies to evolve their operations, increase connectivity, derive real-time insights, improve security, and use AI/ML algorithms to improve efficiency, both edge and cloud computing are becoming increasingly important. Both technologies together allow industries to leverage the benefits of both localized processing and large-scale cloud resources,” Bhargava said.
He added that software technologies such as Kubernetes and Docker provide containerization allowing for easy portability and scalability across diverse computing environments. Management and orchestration systems enable easy deployment, configuration, monitoring, and lifecycle management of such applications across edge devices. AI/ML aided industrial analytics software operates on large datasets and provides deeper insights for more efficient operations.
Impact on smart manufacturing
“By combining the strengths of both edge and cloud computing, industrial facilities can achieve significant technical advantages. In fact, edge computing may be used to extract production data, transform this data so it’s normalized and ready to be consumed, and transport them to cloud resources for a deeper analysis and correlation,” Bhargava said.
Another set of technical advantages comes from the ability to reuse your industrial networking devices as compute resources. Although you could deploy server appliances and span network traffic to them for extracting and processing data, it is much more efficient to embed that processing within the network switches and routers that transport the data. By building your industrial network with equipment that can run custom applications, you can increase scalability and achieve simpler architectures by eliminating the need for standalone appliances.
“Industrial edge and cloud computing are transforming manufacturing into a smarter, more data-driven industry. Some of the common benefits include predictive and preventive maintenance by analyzing sensor data, improved quality control with continuous process monitoring, optimized production processes with AI/ML algorithms, and increased sustainability by monitoring and controlling energy usage,” Bhargava added.
Bhargava said that what makes edge-computing unique and different is how networking devices are now being mobilized as compute nodes, replacing dedicated hardware resources that were commonly implemented for the purpose in industrial networks. By leveraging switches and routers for computing, you not only avoid dedicated resources, but also make your architecture more scalable and simpler, and by removing the need for SPAN networks to send traffic to those resources you also reduce your OpEx.
“Today’s edge and cloud computing are actively playing a role in making industries smarter. Edge and cloud work together, with edge nodes handling real-time processing and initial analysis, while the cloud provides vast storage, advanced analytics, and machine learning capabilities. For example, data can be preprocessed and filtered at the edge before sending it to the cloud, reducing bandwidth requirements and enabling AI applications,” he said.
For example, Cisco provides Cyber Vision, an application that can be loaded into Cisco industrial switches and routers which identifies all connected assets, map communication activities, and assess vulnerabilities through deep-packet inspection (DPI) and active discovery methods. It also continuously monitors assets for any changes in their behavior that could indicate presence of malware. Data from this application can be sent to a cloud portal for further analysis, visualization, correlation, and to determine response.
Another Cisco application allows zero-trust network access (ZTNA) for enforce security policies when remote teams log into industrial assets for configuration, maintenance, or troubleshooting purposes. This is especially useful for geographically dispersed operations.
Industrial Edge and Cloud solutions
Bhargava said that industries across the board can benefit from edge and cloud solutions. The manufacturing sector, for example, can predict failures before they happen and take preventive actions. Real-time decision making can help operations such as autonomous vehicles and robots. Industries like oil and gas or utilities can remotely monitor power generation facilities like wind and solar farms, oil wells, etc.
Cybersecurity is one critical requirement across all industries that stands to greatly benefit from edge and cloud computing. Security applications can now be built into the networking devices, which are in the best position to analyze transiting traffic and derive insights, as explained above.
“Advances in AI can be quite the game changer for many industries. AI algorithms are used to analyze the vast amounts of data generated by IoT devices. Machine learning models can identify patterns and anomalies, enabling predictive maintenance and real-time decision-making. Similarly, AI-powered image recognition is used in quality control processes in manufacturing, as well as in surveillance and security applications in various industries. Natural language processing enables chatbots and virtual assistants to provide human-like interactions,” Bhargava said.
IIoT and Industry 4.0
Bhargava said that “industrial edge and cloud solutions are poised to power the next phase of Industrial IoT and Industry 4.0. For example, edge computing will become more deeply integrated with IIoT devices allowing for faster processing and action on the data generated by sensors and machines, leading to more efficient and responsive manufacturing processes.”
“As connectivity between machines becomes ubiquitous and networks are updated to transport the vastly increasing amounts of data reliably from edge to the cloud in real-time, it will trigger the development of more complex cloud-based solutions that act with physical processes in real time,” he added.
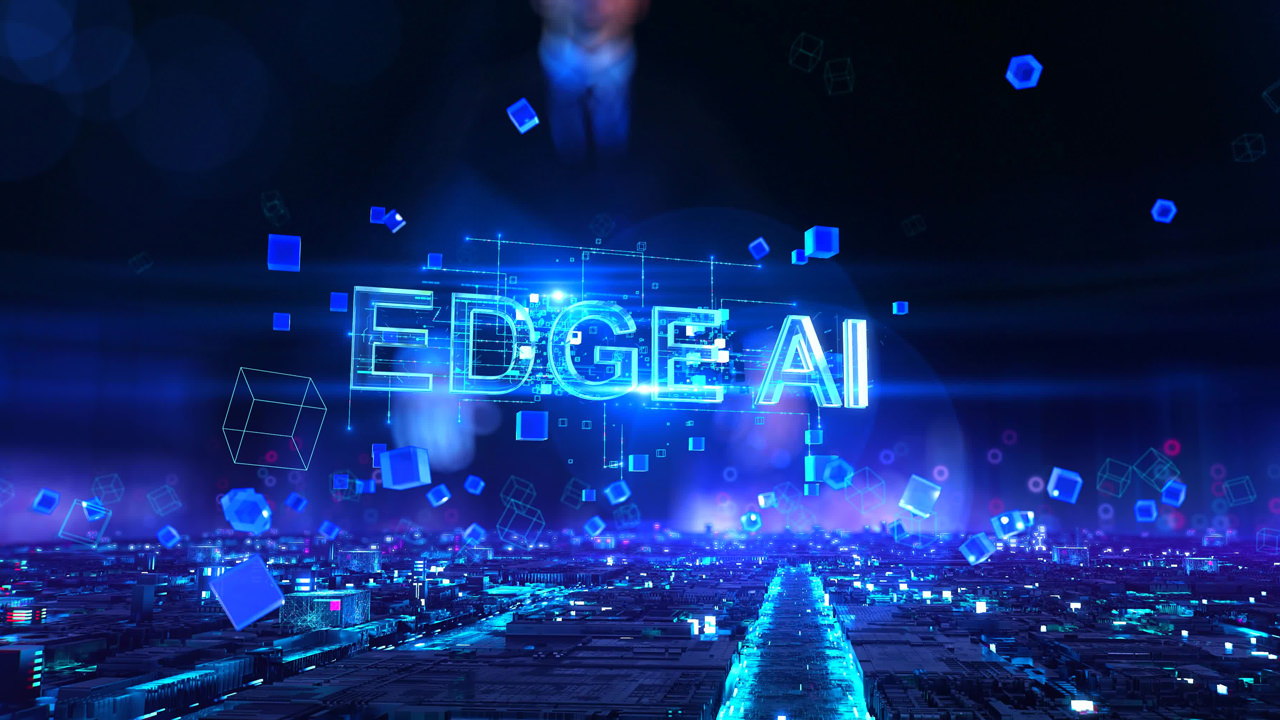
“Artificial Intelligence (AI) is set to transform how things get done in almost every area of business and industrial automation will be a key part of this revolution. While the elevated level of excitement surrounding AI may lead to some short-term disappointment in the initial results, the long-term expectations, if anything, are not high enough,” Steve Fales, Director of Marketing, ODVA.
Impact of Artificial Intelligence
Powerful ability to leverage mathematical and programming models.
Steve Fales, Director of Marketing at ODVA said that “artificial Intelligence (AI) is set to transform how things get done in almost every area of business and industrial automation will be a key part of this revolution. While the elevated level of excitement surrounding AI may lead to some short-term disappointment in the initial results, the long-term expectations, if anything, are not high enough. The emergence of large language models like ChatGPT, that can, in essence, guess with an extremely high degree of accuracy what the appropriate grammar and words should be to answer questions for a chat bot application or to create highly accurate language translations, have proven to be the initial ‘killer app’ showing everyone the value that AI can bring to the table.”
Fales said that one of the key drivers behind AI is that the mathematical and programming models that underpin the technology have been built using open-source libraries and easy to use programming languages like Python. This is enabling AI, machine learning, and deep learning to propagate much faster than would otherwise be possible.
“A data scientist can readily take advantage of the technology to build custom models based on the available open-source libraries that can then be trained either on an open source or a custom data set, that can also be proprietary. Large and small companies alike are racing ahead quickly to create solutions that range from generic tools that allow non-data scientists to use AI to custom built solutions for specific applications,” Fales added.
Technology benefits
Fales said that industrial automation has been leveraging huge amounts of data at very fast speeds to provide responsive and accurate real time application solutions for many decades now. The control algorithms aren’t likely to drastically change any time soon as the loop tuning and controller programs have been optimized over the years by highly skilled engineers. What will change is that the high-level relationships between different devices, machines, applications, and plants can now be better monitored, analyzed, and understood to find opportunities for process improvement. In other words, while micro-level optimization has been done well for some time, macro-level optimization is the new opportunity frontier.
To understand the big picture of a plant, Fales said that it is imperative to look at data differently. While a fast update of data that gets discarded quickly is best for loop tuning, plant wide optimizations will require greater update time intervals and broader sources of data. AI requires large data sets over significant periods of time to identify trends that can then be compared to the current application data to determine both correlation and causality. Additionally, data from seemingly unrelated sources such as the weather, and other time series data that can be scraped from the web, can also provide insights into what is taking place and why. AI models can determine what relationships exist between data from many different sources to help create more accurate predictions. Using large amounts of data from many different sources is referred to as Big Data, which requires using computing capabilities from open-source solutions, such as Hadoop or Apache Spark, to allow the resource intensive calculations to be split up and done across thousands or even millions of servers.
AI creating possibilities
“Processing power and connectivity are the key difference between what is possible today with AI versus in the past,” Fales said. You can now have compute resources spread out over the entire internet and be able to get data from the factory floor to the cloud and back fast enough to allow for near real time optimization.”
An example is that you can now attach vibration, temperature, and sounds sensors to machinery that will allow an AI model to combine this information with other data such as throughput and quality to predict failure. This can help to replace some of the collective knowledge that takes many years to develop but can also be lost very quickly to retirement.
Additionally, industrial communication networks, such as EtherNet/IP, are adopting solutions such as the Process Automation Device Information Model (PA-DIM) and the OPC UA information model to make sure that data from the factory floor can be easily understood and used by cloud applications. The new pathways from the automation device to the edge, cloud, and back also create new security risks. This has led to device level security solutions such as CIP Security for EtherNet/IP, which allows for data encryption and integrity, device authentication, role-based access, device level firewalls, and more.
Newest Industrial Edge and Cloud solutions
“The hardest, costliest, and most dangerous problems are prime opportunities for AI to solve. Machines that breaking down in the middle of the night when support isn’t available, chemical plants where failures can result in environmental disasters, or automotive plant shutdowns that cost millions present compelling business cases to invest the resources to come up with automated models that can help to monitor and proactively solve issues,” Fales said.
One of the limitations of AI today is that it is a complicated art and a science. Data scientists must understand data analytics and programming; however, they also need to understand that the choice of model(s) employed, type of data included in the model, the training data used, and the parameters of the model can be the difference between success and failure. For example, having the right data context and metadata to understand how to best use the information in a model is critical.
Fales said that a lot of pre-work needs to be done to make sure that the data is the most useful. This pre-work can involve tried and true database tools such as SQL, which can be easily connected to industrial networks like EtherNet/IP via solutions from ODVA Member Softing. There isn’t a clear right or wrong answer because there are many methods to creating a successful AI model. Tomorrow, AI models might not be as sensitive to the design considerations as AI may be able to help build the model itself or there may be significant learnings that take place in the future. However, today, AI requires that a custom model be built for a specific application to generate the precise and accurate results needed. Once a model is successfully built and deployed though, it can be used indefinitely.
Looking to the future
“The future for AI on the industrial edge will be realized through embedded AI chipsets and models. As more powerful AI chips are developed and the production costs go down the threshold, the return on investment will lower and allow for increased output and quality,” Fales said.
Additionally, models over time will become less dependent on designer skill, which will lower the cost of implementing AI and thereby increase the usage rate. Furthermore, when AI models become powerful enough to not only be able to handle new situations and data based on an existing model, but to update the model itself based on the new information then we will see a new era of AI in automation and beyond. While we might have to wait some time for self-designing AI to become possible, there are plenty of amazing solutions available today.
For example, new ODVA Member Elementary uses machine learning and EtherNet/IP data to identify quality issues before a product is shipped. The usage of machine learning allows for new issues to be identified that weren’t specifically outlined in an algorithm. In this way, the best of people and machines can be combined, allowing for novel situations to be handled with relative ease while allowing for a production line to go faster than a person could ever handle. With data being analyzed in quantities and at speeds never before possible via AI, resulting in the identification of new trends and information, significant productivity improvements are on the horizon that are poised to pave the way for a new era of prosperity.
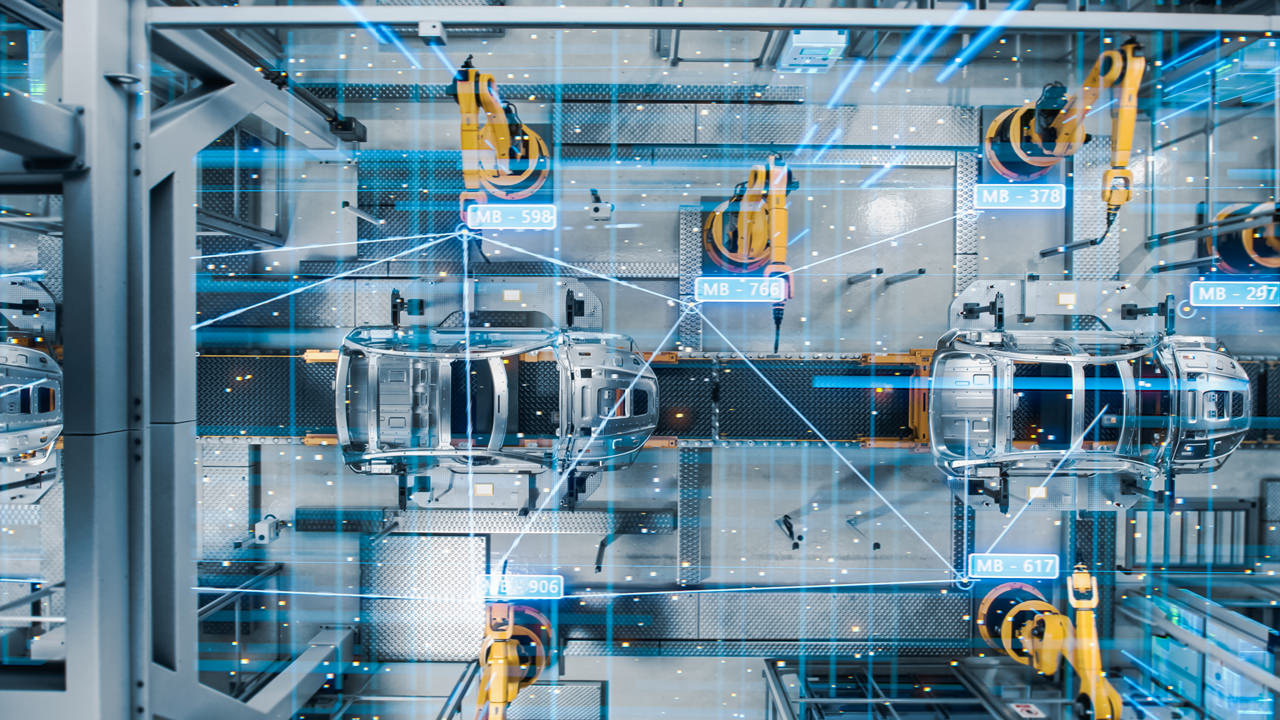
“The future of industrial edge solutions is promising. With all the latest advancements in AI, cloud computing, and data management, the next generation of industry will be more secure and intelligent than ever before,” Collin Brown, Field Application Engineer at Red Lion Control.
Importance of MQTT technology
More streamlined data collection and method for effective interface to the cloud.
According to Collin Brown, Field Application Engineer at Red Lion Control, technology megatrends such as use of MQTT is enabling Industrial Edge and Cloud Computing innovations.
“From a Red Lion perspective, MQTT has been one of the most widely adopted technologies in the cloud computing space. MQTT is a lightweight publish-subscribe, machine to machine network protocol for message queueing. This technology has allowed for more streamlined data collection and provided a simple and affordable method of getting one’s data to the cloud,” Brown said.
“Industries who harness vital data can use MQTT to safely transmit data back to the cloud where it can be stored, interpreted, and visualized. Having all this data in the cloud allows for better predictive maintenance, improved decision-making, and enhanced safety/hazard detection. The introduction of 5G has been essential for the development of these new cloud technologies. 5G offers high bandwidth and low latency, enabling faster data processing and response times. 5G is not just faster than 4G, there is much more to consider. 5G can handle more devices by offering a high capacity for connectivity. With 4G, the connection played as a “one size fits all,” whereas with 5G, depending on the needs of the device, the network energy consumption can be “throttled” for a more efficient output.”
Industrial Edge technology benefits
Brown said that “utilizing an industrial edge in your application simplifies the collection, handling, and analysis of data from industrial devices. This will not only allow insightful decision-making, but also keep IT efforts and costs at a moderate level. Data being computed in the cloud allows for real time information, better record keeping, centralized device management, and remote visibility.”
He added that a well-designed cloud system that monitors itself adds convenience, comfort, and peace of mind. Cloud computing offers several benefits over traditional computing. To start, integrating cloud computing into your application adds scalability. Since there is no longer a need for hardware, companies can expand their IT infrastructure by simply setting up virtual servers. This provides secondary advantages, such as cost reductions, increased flexibility, and improved mobility. Given that cloud computing centralizes assets, collaboration becomes easier for people who may only be able to remotely access data. Utilizing this technology also decreases a company’s carbon footprint since physical servers are no longer necessary.
“One unique modern-day technology that drives cloud computing is virtualization. Virtualization is a technology that creates virtual representations of physical machines, such as servers, storage, and networks, using software that simulates hardware functions. These virtual assets require less construction, less physical materials, and can be created anywhere with an internet connection. In the past, to add machines to your application, you would need to buy a new dedicated device,” Brown said.
This pattern leads to many complications such as device license management, device compatibility, and additional power requirements. With the integration of virtual assets, these headaches quickly go away. These now “software defined” appliances can run on the same platform, taking advantage of the hardware we already own. These virtual assets can be containerized, further adding security to one’s application. If one of the assets needs to be updated, rather than shutting down the entire system, you can simply stop that specific container and proceed with the update process. Moving toward virtualized systems helps keep convenience and security high without sacrificing functionality.
Prime applications/industries
“Industries in remote locations have begun to gain value by making use of cloud solutions. Data that was once stranded locally can now be safely backed up off site. Specifically, the oil and gas industry have strict regulations they must abide by in order to continue operations. With new cloud technology, this indispensable data can now be protected from local memory corruption or loss of connectivity. AI is playing a significant role in the development of new industrial cloud solutions by enabling advanced capabilities that enhance efficiency, productivity, and decision-making,” Brown said.
He added that predictive maintenance has seen huge benefits using AI algorithms to analyze data from industrial equipment, predicting maintenance needs before they occur. Difficult tasks such as energy management can even use AI to analyze consumption and optimize usage. With sustainability being one of the big three facets of Industry 5.0, this technology will speed up meeting sustainability goals without the need for additional manpower. Lastly, AI can be a powerful cyber security tool. Using AI to detect anomalies in real-time leads to faster response times and will overall enhance the cybersecurity of an application.
“The future of industrial edge solutions is promising. With all the latest advancements in AI, cloud computing, and data management, the next generation of industry will be more secure and intelligent than ever before,” Brown said. “Industry 4.0 at its core is based around connectivity. As more industrial edge solutions make their way into applications, industry 4.0 tech will become easier and more affordable for companies to integrate into their day-to-day operations. As the industrial edge evolves, it continues to focus on these key factors that can be the make-or-break for companies who chose to add this tech to their systems.”
As we start to see this tech work successfully, there will be more demand. More demand leads to more money and time invested in moving this technology forward, resulting in better cybersecurity and resource management.
“All these factors come together to create an industrial edge that helps companies not only collect data from their applications, but also get the most use from it. Data is no longer trapped at the ground level, with these new advancements, we will start to see data being further accessed, connected, and visualized,” he added.