TechnologyNovember 20, 2024
Tackling AI processing challenges at the Industrial Edge
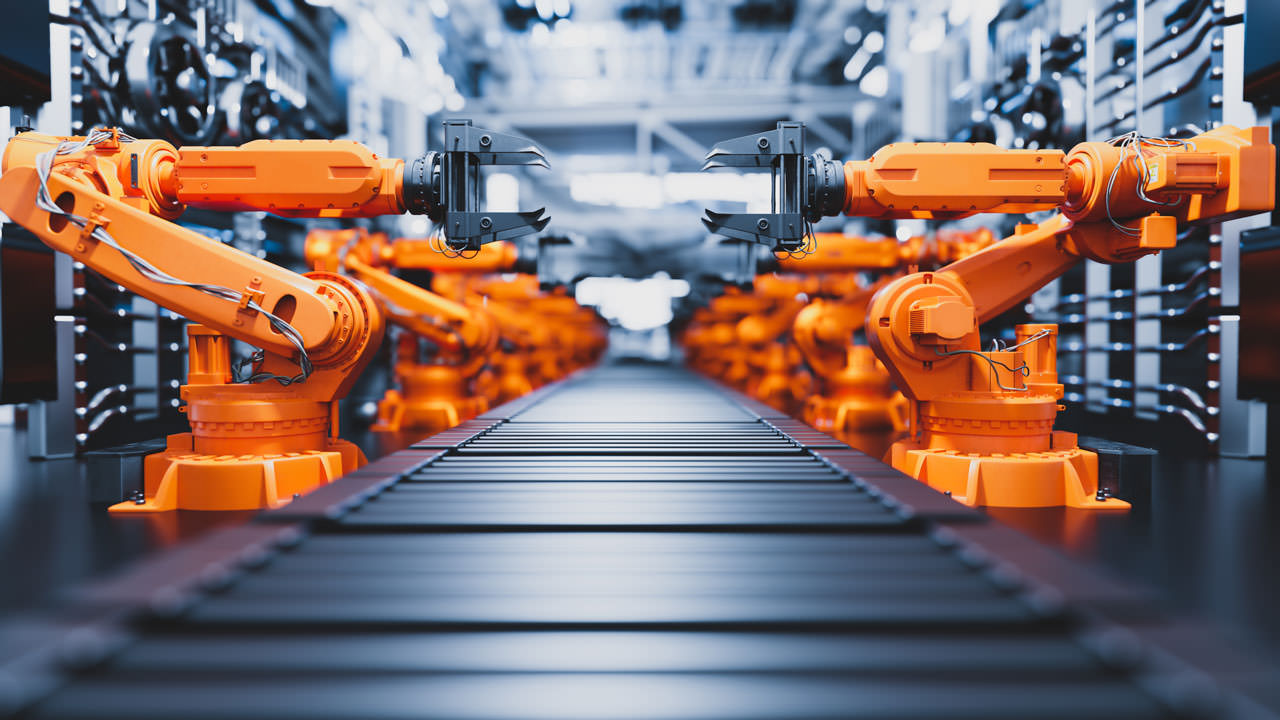
The challenges industrial environments face when processing AI data at the edge can include cost and power constraints, as well as limited memory resources. While cloud solutions can accommodate higher costs and power requirements, edge applications often operate within stricter financial and energy limits.
As Artificial Intelligence (AI) technology shifts from centralized cloud systems to ‘the edge,’ industrial applications face major hurdles in processing AI data. A key challenge is balancing AI performance with power efficiency, particularly for demanding tasks like Generative AI, where high performance demands often clash with energy constraints. Real-time processing is crucial for industries needing timely data-driven decisions, but doing so with limited resources remains a significant obstacle.
For instance, applications such as autonomous vehicles (drones, cars, industrial robots), where instantaneous decision-making is essential–any delay in data processing could have dire consequences. Additionally, integrating new AI technologies with existing architectures and legacy systems often poses significant technical, cost, and institutional hurdles. Expanding AI applications to edge environments can also increase exposure to cyber threats.
When AI processing relies on continuous cloud communication due to limited edge capabilities, it increases the risk of exposing critical data, highlighting the importance of robust security measures. AI platforms capable of in-system processing without relying on cloud communication are therefore more desirable, offering lower latency and higher levels of safety and security.
Finally, challenges with AI applications at the edge can include cost and power constraints, as well as limited memory resources. While cloud solutions can accommodate higher costs and power requirements, edge applications often operate within stricter financial and energy limits, making lower-cost, energy-efficient AI processing crucial.
Furthermore, the memory capacity at the edge is limited compared to cloud systems, necessitating efficient use of available memory to maintain high performance. Physical space constraints further complicate matters, as edge devices must fit within compact environments such as drones, 5G base stations, manufacturing robots, or aerospace solutions.
Overcoming these challenges
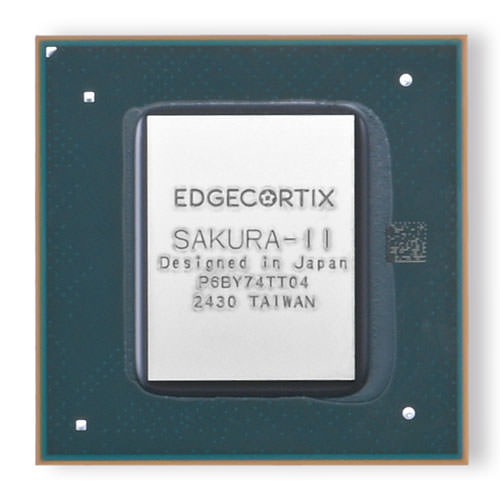
SAKURA-II is designed for applications requiring fast, real-time Batch=1 AI inferencing with excellent performance in a small footprint.
EdgeCortix addresses these challenges with innovative solutions. The company’s SAKURA-II AI accelerator platform is designed to deliver near cloud-level AI performance while drastically improving energy efficiency, making it exceptionally suitable for demanding edge workloads. Additionally, the Dynamic Neural Accelerator® (DNA) architecture provides runtime reconfiguration, optimizing data paths for efficiency while providing real-time processing capabilities, which ensures low latency—a key requirement for edge applications.
The MERA Compiler Framework facilitates the deployment of AI models in a framework-agnostic manner, supporting seamless integration with existing systems and diverse processor architectures. EdgeCortix also offers modular AI accelerator solutions, such as PCIe Cards and M.2 Modules, which seamlessly integrate into existing systems to streamline AI deployment timelines.
Real-world applications
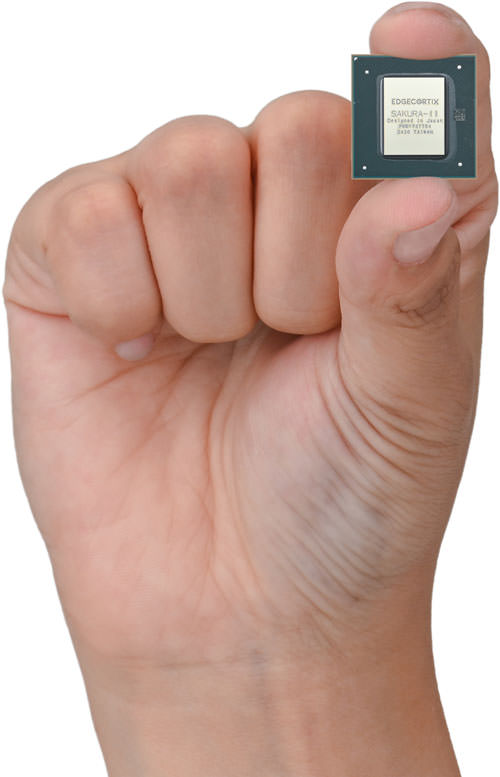
AI has many applications in sorting through volumes of big data coming from manufacturing floors today. At the edge, higher speed, higher resolution sensors including video reveal more about processes and product quality than ever.
Real-world deployment examples illustrate the effectiveness of these solutions. In smart cities, EdgeCortix technology can enhance AI capabilities for traffic management and security monitoring by processing large volumes of data from cameras and sensors in real-time. Additionally, edge AI solutions can improve public safety by enabling high-resolution video analysis in crowded areas and enhance emergency response with accurate recognition of people and objects.
In manufacturing, edge AI solutions can optimize production lines, predict equipment failures, and improve quality control through immediate sensor data analysis, improving overall efficiency and reducing downtime. In the aerospace industry, these solutions can monitor aircraft engine performance and predict component wear, contributing to safety and reliability while lowering maintenance costs.
Overall, as AI continues to proliferate across various industries, the challenges faced by embedded designers transitioning from cloud to edge solutions become increasingly necessary and significantly more complex. Selecting the correct AI hardware solutions platform and provider is crucial in order to overcome these obstacles and successfully implement edge AI products. By evaluating AI accelerators, like EdgeCortix’s SAKURA-II, against the key design challenges outlined, engineers can develop energy-efficient and high-performing solutions that meet the demands of modern applications within existing systems.
This shift toward edge AI not only enhances real-time data processing capabilities but also supports the growing need for cost-effective and scalable AI technologies across sectors such as smart cities, manufacturing, telecommunications, aerospace, and others. With the increased demand for Generative AI processing at the edge, it is critical for AI solutions to operate these complex, multi-billion parameter models efficiently with very low power consumption.
SAKURA-II meets these generative AI requirements using DNA architecture, optimizing data paths, and employing parallelized processing for maximum efficiency.
These advancements demonstrate EdgeCortix’s ability to tackle the critical challenges of AI data processing at the edge across various business sectors. They also illustrate how EdgeCortix solutions are engineered to provide superior performance and power efficiency for edge AI applications.