TechnologyJanuary 23, 2025
Artificial intelligence in smart manufacturing
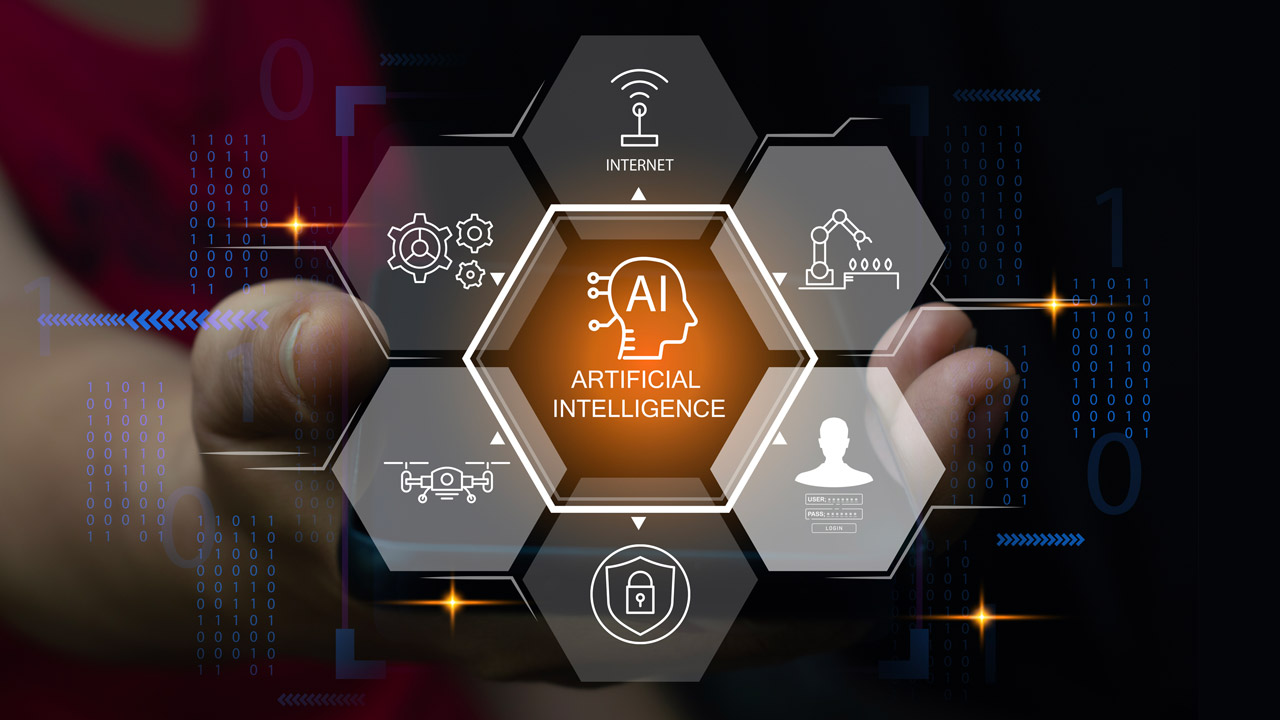
AI technology is helping to empower automation and control engineers by automating routine tasks, improving system efficiency, and enabling smarter decision-making. This allows engineers to focus on innovation and higher-value activities, ultimately driving better outcomes for manufacturing operations
Artificial intelligence solutions are poised to make an impact in manufacturing by providing tools for AI-enabled digital transformation including machine learning, advanced analytics, cloud operating models and use of neural networks.
For this special report on AI in manufacturing, IEB reached out to industry experts to get their perspective on the technologies that are impacting smart manufacturing operations.
Industry leaders have responded with their take on the latest trends and how AI will create agile, efficient, and sustainable manufacturing ecosystems. It will enable industries to address challenges like rising consumer expectations, supply chain complexities, and environmental concerns, ultimately driving innovation and competitiveness.
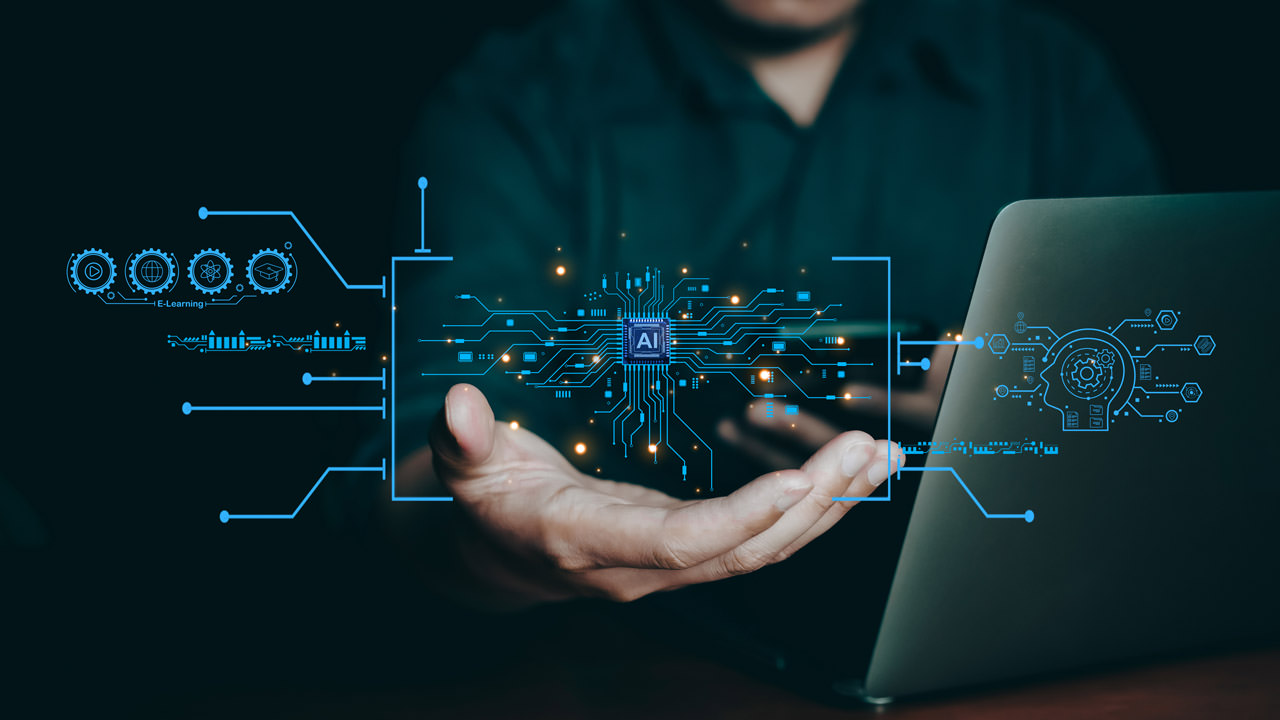
“AI technologies and megatrends are set to reshape manufacturing, driving Digital Transformation by creating smarter, connected, and more autonomous systems. By 2025 and beyond, AI-powered analytics will leverage IoT and edge computing to process real-time data, enabling predictive maintenance, optimized production, and streamlined supply chains.” — John Marchiando, Industrial IoT Manufacturing Lead – Americas, Cisco.
AI-driven analytics spur digital transformation
Reducing downtime, increasing efficiency and enhancing decision-making.
According to John Marchiando, Industrial IoT Manufacturing Lead – Americas, and David Gutshall, Senior Manufacturing Sales Leader at Cisco, AI is poised to impact manufacturing operations using AI-powered analytics and edge computing.
“AI technologies and megatrends are set to reshape manufacturing, driving Digital Transformation by creating smarter, connected, and more autonomous systems. By 2025 and beyond, AI-powered analytics will leverage IoT and edge computing to process real-time data, enabling predictive maintenance, optimized production, and streamlined supply chains. These capabilities will reduce downtime, increase efficiency, and enhance decision-making,” Marchiando told the Industrial Ethernet Book recently.
“Advanced robotics and autonomous systems will enable greater flexibility in manufacturing, allowing rapid adaptation to product variations and fluctuating demand. AI-driven automation will support customization at-scale, while maintaining efficiency and precision,” Marchiando said. “Digital twins, combined with AI and real-time data, will revolutionize process simulation, allowing manufacturers to optimize workflows, test innovations, and accelerate time-to-market without disrupting operations.”
He added that sustainability will become a central focus, with AI improving energy efficiency, waste reduction, and resource utilization. Manufacturers will adopt AI-enabled tools to meet environmental regulations and achieve sustainability goals while enhancing cost-efficiency.
AI-driven megatrends
Gutshall added that emerging megatrends such as 5G and the slow-to-develop industrial metaverse will further enhance connectivity, enabling seamless communication across devices and systems while supporting immersive, remote collaboration. These technologies will empower manufacturers to operate with unprecedented agility and resilience, even amid global disruptions.
“AI and related megatrends will create agile, efficient, and sustainable manufacturing ecosystems,” Gutshall said. “They will enable industries to address challenges like rising consumer expectations, supply chain complexities, and environmental concerns, ultimately driving innovation and competitiveness in the era of Industry 4.0 and beyond.
While typical applications provide static, rule-based capabilities, AI offers more dynamic, data-driven, and potentially solutions that are self-improving. It turns your manufacturing systems into intelligent, adaptive ecosystems that can respond to challenges and opportunities far more effectively than in the past. The results are greater efficiency, improved quality, reduced costs, and a significant competitive advantage for those implementing this technology.
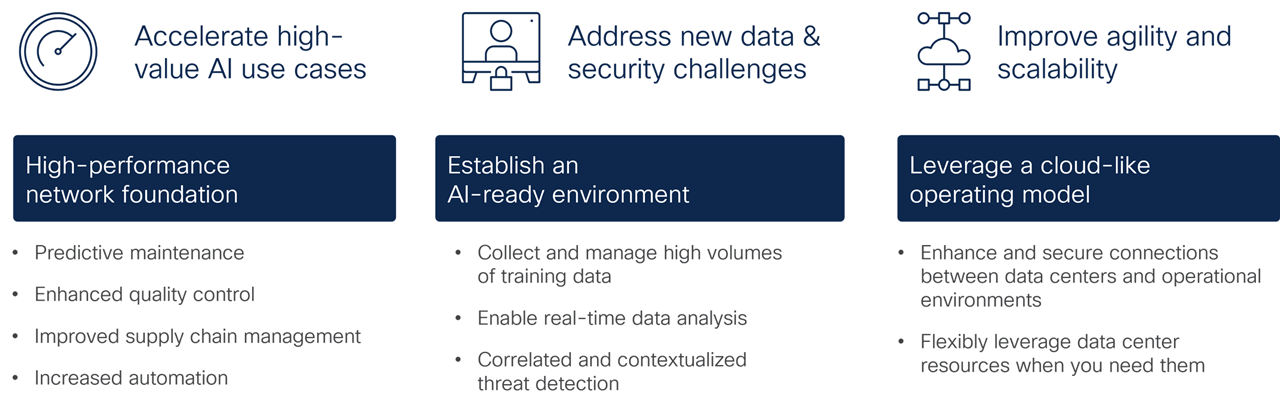
Realize the promise of AI more quickly with advanced technology solutions that address networking, security and operational challenges.
AI in manufacturing
Marchiando and Gutshall said that AI solutions in smart manufacturing offer distinct advantages, enhancing efficiency, precision, and adaptability.
AI’s predictive maintenance, analyzing sensor data to forecast failures and reduce downtime, is a notable application. Machine learning optimizes processes, improving energy efficiency and reducing waste. AI-driven computer vision enhances quality control with improved defect detection and real-time feedback.
Advanced AI models forecast demand and manage inventory, optimizing levels and adapting to market trends. AI-powered robots adapt to task variations and support human collaboration, unlike rigid pre-programmed robots.
AI enables quick reconfiguration for new products, facilitating mass customization. Digital twins simulate and optimize systems in real-time, surpassing static simulation models.
Supply chain optimization, with AI’s logistics optimization and disruption adaptation, enhances efficiency and costs. AI also aids workers with existing augmented reality (AR) tools and adaptive training, boosting productivity and reducing errors.
IoT integration, processing real-time data at the edge, enables faster and smarter decision-making.
Overall, AI transforms manufacturing with smarter, autonomous systems that enhance productivity, reduce costs, and adapt to complex demands.
They added that AI impacts manufacturing by enhancing efficiency, quality, and flexibility. Notable examples include:
- Predictive Maintenance: AI sensors predict equipment failures, minimizing downtime and costs.
- Quality Control: AI-driven vision systems detect defects and analyze quality, ensuring consistency.
- Process Optimization: AI adjusts production parameters to minimize waste and optimize performance.
- Supply Chain Management: AI optimizes inventory, demand, and logistics, improving efficiency.
- Worker Assistance: AI monitors work environments and supports operators, boosting productivity.
- Flexible Manufacturing: AI enables agile systems to meet personalized product demands, allowing for adaptability.
AI has the potential to revolutionize industries by enhancing efficiency, productivity, and adaptability. It can assist in optimizing processes, identifying ways to reduce waste, and lowers operational costs while ensuring consistent quality and minimizing defects. AI-driven automation enables faster production cycles and customization at-scale, supporting greater ability in meeting market demands. Predictive capabilities reduce downtime and enhance resilience by anticipating equipment failures and supply chain disruptions.
AI also fosters innovation through advanced simulations, data-driven R&D, and rapid prototyping, accelerating breakthroughs in product design and processes. By augmenting human capabilities, AI shifts workforce focus to high-value tasks, driving upskilling and transforming roles. AI also supports sustainability goals by reducing resource consumption and emissions, helping companies meet regulatory requirements. These advancements provide a significant competitive advantage, reshaping industry dynamics and preparing businesses for a more sustainable, future-ready landscape.
AI solutions
“AI solutions help to empower automation and control engineers by automating routine tasks, improving system efficiency, and enabling smarter decision-making. This allows engineers to focus on innovation and higher-value activities, ultimately driving better outcomes for manufacturing operations,” Marchiando said.
Some examples of these implementations are in areas like complex data management, where the growing volume of IoT and sensor data can be overwhelming. AI can assist engineers in processing this data in real time and then delivering actionable insights to simplify decision making.
“Engineers often struggle with identifying and diagnosing system faults quickly, especially in complex systems. AI-powered analytics can detect anomalies, pinpoint root causes, and suggest corrective actions, reducing downtime and troubleshooting time,” Gutshall added.
Another example is around scalability and adaptability. Engineers can face difficulties reconfiguring systems for new products or workflows. AI enables flexible automation, learning and adapting to the new conditions without extensive reprogramming.
“AI can assist automation and controls engineers to improve sustainability by managing energy consumption. AI can help analyze usage patterns and recommend optimizations to processes to reduce waste and cost,” Marchiando said.
“Modern systems have numerous interconnected variables, making manual control and optimization difficult. AI excels at managing complex, nonlinear relationships, helping engineers maintain optimal performance across the entire system,” he added.
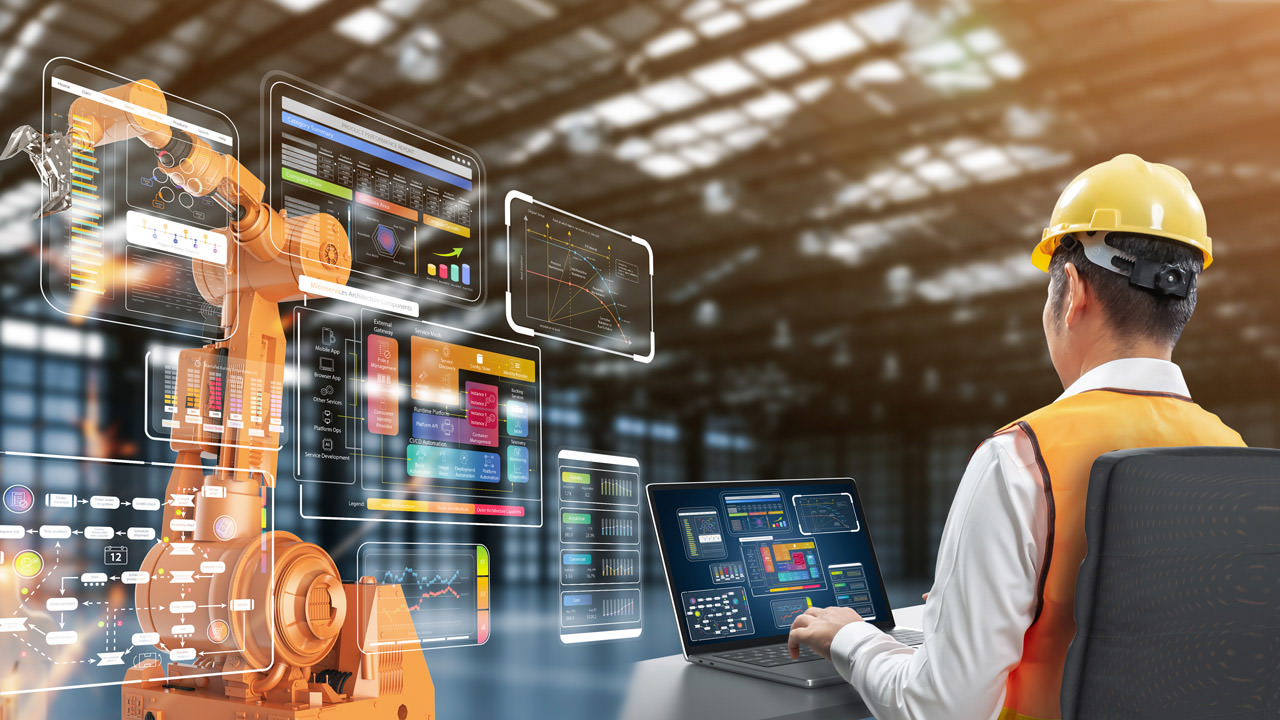
“We are already seeing AI-powered systems enabling predictive maintenance, optimizing production workflows, and automating quality control and defect detection on the shop floor. AI-driven insights from connected devices and processes allow manufacturers to make faster, more informed decisions to boost efficiency, quality, and sustainability,” Dr. Matthias Loskyll, Senior Director Software, Virtual Control and Industrial AI, Siemens AG.
AI-enabled digital transformation
Manufacturing companies to increasingly leverage AI capabilities.
Dr. Matthias Loskyll, Senior Director Software, Virtual Control and Industrial AI at Siemens sees AI as playing a key role in the continuing development of customized, flexible production systems.
“As a global leader in industrial automation and digitalization, we at Siemens see AI and generative AI technologies playing a pivotal role in accelerating the digital transformation of manufacturing. Key megatrends like the rise of the Internet of Things, the growing importance of data analytics, and the increasing demand for customized, flexible production will drive manufacturers to increasingly leverage AI capabilities,” Loskyll said.
He added that reaping AI’s benefits in industry is complex due to stringent standards, critical reliability requirements, and a shortage of skilled experts. It must meet the rigorous requirements and standards of the most demanding industrial environments. AI in industry has to be industrial-grade. Siemens is committed to making industrial-grade AI accessible without the need for specialized AI expertise, enabling companies to drive transformation effectively.
“We are already seeing AI-powered systems enabling predictive maintenance, optimizing production workflows, and automating quality control and defect detection on the shop floor. AI-driven insights from connected devices and processes allow manufacturers to make faster, more informed decisions to boost efficiency, quality, and sustainability. Additionally, AI will enable more personalized, on-demand production to meet evolving consumer preferences. In production, we’re already harnessing the advantages of AI, and its future potential promises to revolutionize efficiency, precision, and innovation on an unprecedented scale,” Loskyll said.
Benefits of AI solutions
Compared to typical automation and analytics applications in use today, Loskyll said that AI-powered systems offer far greater capabilities to sense, analyze, and optimize manufacturing processes in real-time. For example, AI-enabled machine vision can detect defects and anomalies with superhuman accuracy, going beyond the limitations of traditional rule-based quality inspection.
Similarly, predictive maintenance AI models can forecast equipment failures before they occur, allowing for proactive maintenance to minimize unplanned downtime. AI also enables the dynamic optimization of production parameters, supply chains, and logistics to continuously improve efficiency, quality, and throughput. Importantly, the self-learning nature of AI means these systems continually enhance their own performance over time, adapting to changing conditions on the factory floor. This level of adaptive intelligence simply cannot be achieved with conventional software applications.
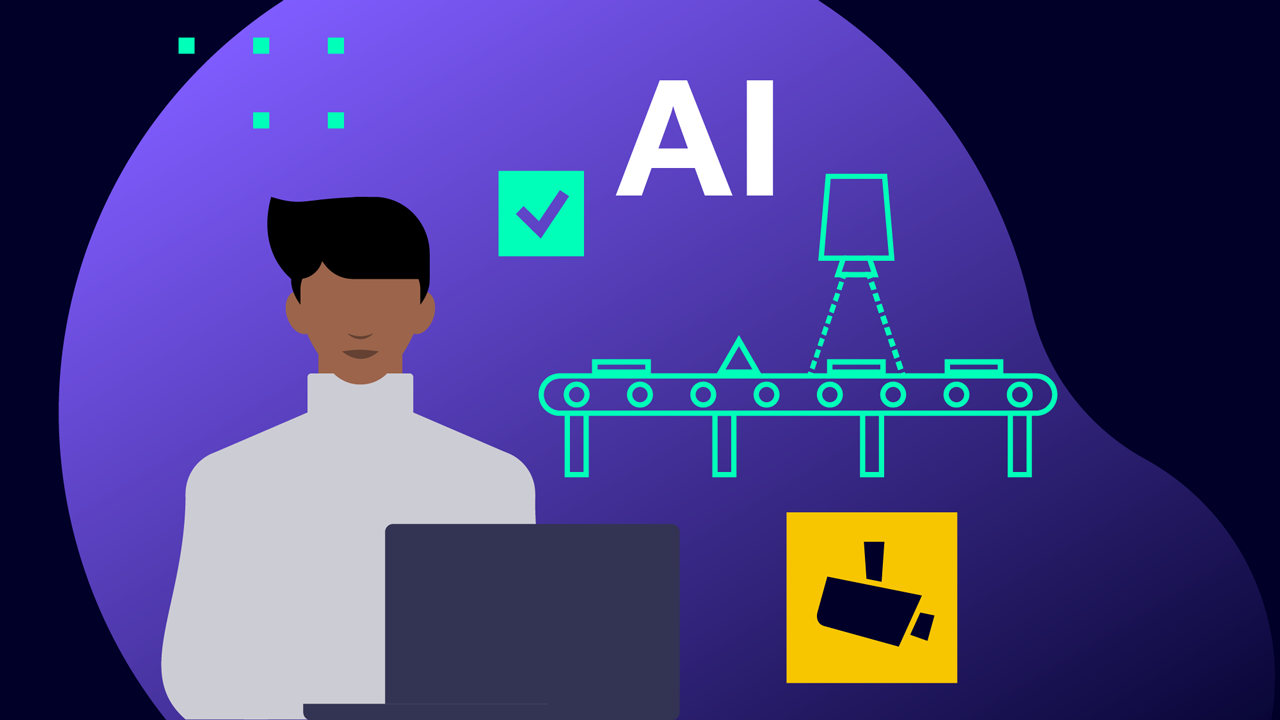
Siemens’ Inspekto – ready-to-use visual inspection.
Impacting the production lifecycle
AI-powered systems are delivering significant benefits across the production lifecycle. In quality control, AI-enabled machine vision can detect defects with speed and accuracy, far exceeding human inspection capabilities. This allows manufacturers to catch issues earlier, reduce waste, and improve overall product quality. For example, in the automotive industry, manufacturers must avoid scratches, dents, poor welds, and faulty electronic components.
The system learns the “good” parts and then detects the anomalies and defects, even if they are not predefined. Siemens’ Inspekto is an automated, easy-to-use visual inspection solution that uses AI but doesn’t require AI or vision expertise to set up and use. It delivers a versatile, easy-to-deploy inspection solution that adapts to changes in the production line and requires no machine vision expertise. It also requires only a small amount of production data of only twenty good samples, which makes it an easy entry point for companies who want to use AI-based machine vision for their quality inspection needs.
“Data-driven condition monitoring of an entire plant or multiple sites is challenging, and manual analysis is too complicated and time-consuming. AI systems can provide maintenance workers with the equivalent of an experienced expert, continuously analyzing all the data from machines, such as temperature, vibration, torque, and speed,” Loskyll said.
“As a result, AI can detect anomalies, alert human workers, and provide actionable insights based on past events. In addition to facilitating data analysis, AI can also provide early warning signs of when equipment is likely to fail and recommend specific actions to prevent those failures.”
He added that these algorithms are continuously trained with new data and insights, so they get better over time. Siemens’ Senseye Predictive Maintenance is an AI-based solution that automatically generates machine and maintenance behavior models to direct attention and expertise where it’s needed most. It integrates with any asset, system or data source, using data that has been already collected or newly installed sensors, and provides generative AI capabilities that make predictive maintenance more conversational and intuitive.
Adaptive process control
One key challenge is the need for more robust, adaptive process control. Traditional control systems rely on rigid, rule-based algorithms that can struggle to account for the variability and complexity of real-world manufacturing environments. In contrast, AI-driven control systems can learn from sensor data, adapt their parameters in real-time, and optimize production outputs based on dynamic conditions. This allows for tighter process control, improved quality, and greater flexibility.
Another major challenge is the difficulty of predicting equipment failures and anomalies. Unplanned downtime remains a major source of lost productivity and revenue for manufacturers. AI-powered predictive maintenance solutions can analyze sensor data patterns to forecast issues before they occur, enabling proactive maintenance and maximizing asset uptime.
“Automation and control engineers also face the ongoing struggle to extract meaningful insights from the vast amounts of data generated across production systems,” Loskyll said. “AI-based analytics can uncover hidden correlations, identify optimization opportunities, and generate predictive models that would be virtually impossible for human analysts to detect.”
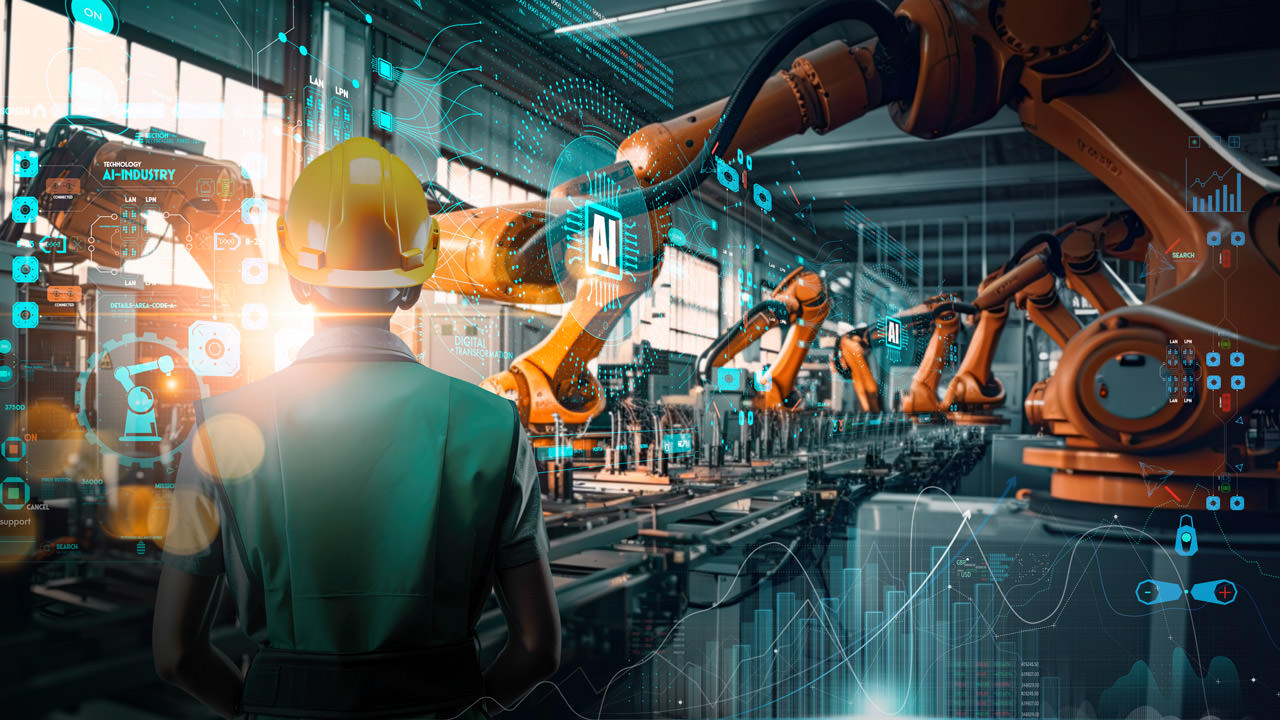
“AI will play a critical role in shaping and enabling digital transformation in manufacturing through 2025 and beyond. AI will drive advances in quality control and supply chain optimization. The integration of AI with IoT and big data analytics will enable real time monitoring and decision making, leading to increased efficiency and reduced downtime,” Dr. Christoph Kelzenberg, Chief Digital Office, Director Digital Innovations & Director Operational Excellence at Phoenix Contact.
AI-powered automation
Advances in quality control and supply chain optimization.
According to Dr. Christoph Kelzenberg, Chief Digital Office, Director Digital Innovations & Director Operational Excellence at Phoenix Contact, AI will help integrate IoT communications with Big Data analytics into AI-powered automation.
“AI will play a critical role in shaping and enabling digital transformation in manufacturing through 2025 and beyond. AI will drive advances in quality control and supply chain optimization. The integration of AI with IoT and big data analytics will enable real time monitoring and decision making, leading to increased efficiency and reduced downtime,” Kelzenberg said.
“In addition, AI-powered automation will improve production processes and enable greater flexibility and adaptability. These technologies will also facilitate the development of smart factories, where networked systems and machines can communicate and collaborate seamlessly,” he said.
Technology benefits
Kelzenberg said that AI solutions offer a wide range of benefits for smart manufacturing that go beyond what is possible with typical applications today. AI can improve quality control by identifying defects and anomalies in real time, resulting in higher product quality and less waste. In addition, AI-powered supply chain optimization can streamline operations, shorten lead times and improve inventory management.
“AI solutions are being used across the manufacturing industry in a variety of ways. For example, AI-driven robots and automation systems are used for tasks such as assembly, welding and solder to improve precision and efficiency,” Kelzenberg said. “AI-driven predictive maintenance systems monitor the condition of equipment and predict failures before they occur, minimizing downtime. The positive effects of these developments are higher productivity, lower operating costs and improved product quality. AI solutions make it possible to innovate and develop new products faster i.e. the design and creation of circuit diagrams.”
Application challenges
Kelzenberg added that one of the most important challenges is the need for data analysis and decision-making in real time. AI can process large amounts of data quickly and accurately and deliver actionable insights.
Another challenge is maintaining plant reliability and minimizing downtime. In addition, AI can optimize control systems, improve efficiency and reduce energy consumption. These solutions make it possible to focus on more strategic tasks and drive continuous improvement.
Leveraging data in manufacturing
An all-purpose AI can solve a wide range of production problems.
Steve Mustard, an independent automation consultant and subject-matter expert for the International Society of Automation (ISA), said that while we are still not able to realize the possibilities of artificial general intelligence, an all-purpose AI that can solve wide ranges of disparate problems, AI is already having a major impact in manufacturing.
“The use of generative AI to generalize information and make predictions is a major use case. Deep learning can be applied to automated inspection systems, analyzing images, video, and other sources of data to identify patterns that predict manufacturing issues or equipment failure,” Mustard said. “The internet of things (IoT) and smart sensors provide vast amounts of data that AI systems analyze to optimize and control processes in real time.”
Impact of AI
Mustard said that AI solutions allow manufacturers to analyze very large data sets and identify patterns and relationships that were previously invisible to existing methods. AI solutions can also provide more repeatable and consistent results and only tend to improve as more data is analyzed.
Generative AI enables manufacturers to mine vast quantities of standards, policies and procedures to improve requirements development, compliance assessment and standard operating procedure production.
Deep learning can be used in image, video and speech recognition, data visualization and trend analysis in automated inspection systems, predictive maintenance and quality control.
Industry applications
“Autonomous and flexible robots, including collaborative robots (cobots) are incorporating AI-based vision-language-action models, enabling them to more closely mimic human capabilities in perception, understanding, and response to their operating environment,” Mustard said.
AI is also being used in digital twins to create real-time system simulations that can be compared to real-world systems for control, diagnostics and prediction, significantly enhancing operational efficiency, reducing risks, and supporting smarter, more proactive decisions.
He added that some of the ongoing challenges that automation and control engineers face that AI can address include:
Decision making: with complex environments, large volumes of data and many interdependencies, AI can support engineers with better and more reliable, repeatable, analysis.
Efficient use of time: AI can help engineers shift their focus to more higher value tasks by supporting the processing of large amounts of data, for instance validating standards, policies, procedures and producing compliance reports.
Detecting cybersecurity threats: cybersecurity is a major risk that automation and control engineers must manage. Having the ability to analyze network traffic and end point activity and identify potential threats early is a major benefit.
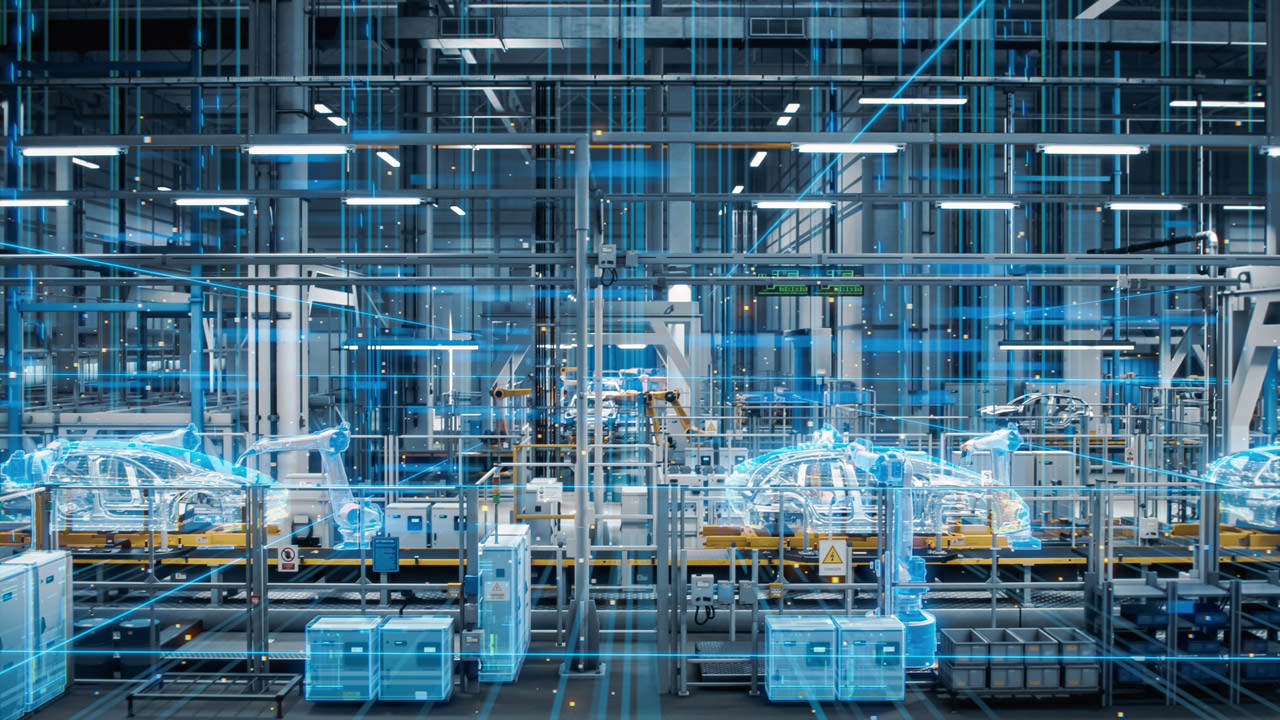
“AI provides the opportunity to continue to reshore manufacturing due to the ability to automate lower value add tasks and enable higher worker productivity. While “dark factories” that don’t need lights due to complete automation aren’t likely to become commonplace anytime soon, AI will help to solve more problems than previously possible to reduce defects and increase total output,” Steve Fales, Director of Marketing at ODVA.
The power of neural networks
AI technology will enable new era of digital transformation in manufacturing.
Steve Fales, Director of Marketing at ODVA said that Artificial Intelligence (AI), machine learning, and deep learning are often used interchangeably in high level business discussions. The distinctions are important though to better understand how neural networks will enable a new era of digital transformation in manufacturing.
“
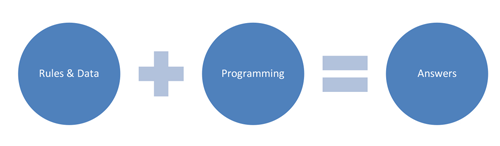
Programming Paradigm.
At the highest level, AI is the process of automating complicated tasks that normally require the intellectual capability of a person. The first subset of AI is machine learning, which is the idea that machines can learn beyond their original programming. Previously, rules and data were used with programming to create answers,” Fales told the Industrial Ethernet Book recently.
“Now it is possible to use data and answers with machine learning to create rules to predict answers from data. The next subset of AI and machine learning is deep learning, which is simply adding multiple layers of transformation between the original input and the final output. Deep learning is what is allowing powerful neural network models to solve highly complex problems in industrial automation today,” Fales said.
Leveraging “deep learning”
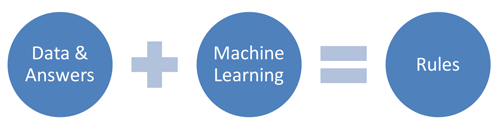
Machine Learning Model.
Fales said that one of the biggest hurdles to leveraging deep learning in plants is labelled data. The internet has enabled large language models to access huge quantities of books, questions and answers, and text conversations as well as labelled pictures of things such as people, animals, and food. However, there doesn’t currently exist a free storehouse of manufactured products being labelled with various errors.
This means that manufacturers will have to create their own graphic libraries with metadata describing the product, any errors, and other pertinent information. To help overcome the comparatively low number of data points, deep learning training techniques can be used such as splitting the same smaller data set into multiple partitions. This can allow the same data to be organized into different ways to mimic being a larger data set by using different slices of the same data for outcome validation and different slices for model training per the Data Folding Example.

Data Folding Example.
Deep learning models are open source and available for use by anyone with expertise in both data modeling and” system design,” Fales said. “The challenge is that model selection is both an art and a science that requires extensive mathematics, programming, and data modeling expertise. To solve that problem, leaders in industrial automation are creating software tools that enable controls engineers to upload datasets, add labels, and identify the problem to be solved.”
He said that the backend of the software then leverages the proper deep learning techniques to create a local, custom model that can provide an ongoing application solution. An example of this is Rockwell Automation’s FactoryTalk LogixAI that allows users to leverage their existing EtherNet/IP control data for problem solving such as predictive maintenance. A significant advantage of using private AI solutions is that proprietary data is then blocked from being incorporated into a larger public model for others to be able to access.
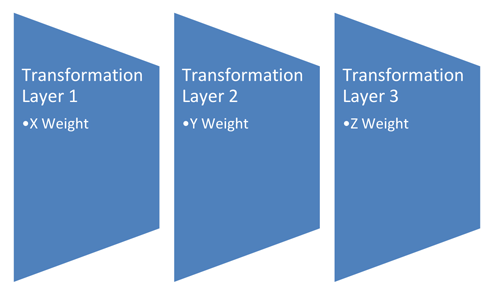
Neural Network Transformation Layers.
AI in general can seem to be too complicated since many different deep learning models, layers, and weights are used, or even lacking in meaning with over usage of the latest buzzword. What’s important to understand is that deep learning is simply pattern recognition leveraging existing mathematical principles such as matrices for images, linear regression, and other functions to achieve the best fit to the data. Deep learning breaks large problems such as identifying whether an automotive body weld is defective into much smaller problems such as whether properly done weld rings, excess material, and/or gaps lacking material are identified. Another common example is facial recognition where the problem gets broken down into hundreds or thousands of data points such as distance between eyes, eye shape, eye color, eyelash angle, nose length, etc.
Basic neural network models work by transforming the original data set into much simpler and more homogenous data that can allow the mathematical functions to search for best fit curves. Neural networks use multiple transformation layers with weights applied to each layer. The next step is to make predictions that are compared to the actual data. The difference between the predictions and the actual data nets a loss score. The weights can then be changed via an optimizer to improve the loss score in an iterative process. It’s important not to optimize the model too much though or the model will start to become too dependent on the training data. Overfitting a model to the training data makes it too specific and not as generally useful for predicting outcomes with new data.
AI in manufacturing
“AI is being used today in applications such as recognizing whether workers are wearing appropriate Personal Protective Equipment (PPE) for the hazards in each environment. Workers not wearing a hard hat, safety glasses, reflective vests, respirators, etc. can be identified and flagged automatically to prevent an accident before it happens. Additionally, AI can automatically identify defects such as improperly manufactured cable connectors to improve quality and increase throughput,” Fales said. “AI can even operate highly complex machinery that takes years of training for a human operator. To increase productivity, people need force multipliers to help them to be able to do more with less. AI can enable factory workers to be safer, more productive, and to focus more intellectually engaging higher value add tasks. AI will supercharge the capability of workers in 2020s and 2030s in the same way that personal computers did in the 1990s and 2000s.”
Fales said that, to best take advantage of AI going forward, it’s more important than ever to adhere to the fundamentals of proper network design and to rely on data models. The creation of separate zones of controllers, switches, and devices is a well-known security technique that is also valuable when leveraging AI at the edge since it will help to minimize network propagation and ensure traffic stays within the limits of bandwidth. Data models that include semantic and scale information, so that control data can be easily ingested by AI tools, are also a hugely valuable investment. Examples of this include OPC UA for discrete automation and PA-DIM for process automation that can both be taken advantage of by industrial Ethernet networks such as EtherNet/IP.
Engineering challenges
AI provides the opportunity to continue to reshore manufacturing due to the ability to automate lower value add tasks and enable higher worker productivity. While “dark factories” that don’t need lights due to complete automation aren’t likely to become commonplace anytime soon, AI will help to solve more problems than previously possible to reduce defects and increase total output.
If you’ve visited a plant recently, you’re already aware of the importance of safety given the preparatory safety videos, usage of PPE, and limited areas that guests can access. Total Recordable Incident Rate (TRIR) is a US Occupational Safety and Health Administration metric that is a key factor in determining whether to do business with another organization. AI can help to automate dangerous tasks and to therefore lower TRIR scores and reduce potential liability.
“One of the challenges of AI that is starting to come to the forefront is its impact on sustainability. AI uses more energy than a traditional Google search engine search by approximately a factor of ten, according to Google generative AI,” Fales said. “While optimization of AI will hopefully narrow that gap soon, the usage of more sustainable energy sources such as nuclear, solar, and wind can help to mitigate those concerns. It’s also important to make appropriate decisions of onboard, edge, or cloud AI based on cybersecurity threat assessments and business needs. While the benefits of AI clearly outweigh the downsides, it’s important to understand the pros and the cons to appropriately deploy this new technology in the correct applications.”